LAPSE:2023.31414
Published Article
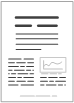
LAPSE:2023.31414
A Short-Term Load Forecasting Model Based on Crisscross Grey Wolf Optimizer and Dual-Stage Attention Mechanism
April 18, 2023
Accurate short-term load forecasting is of great significance to the safe and stable operation of power systems and the development of the power market. Most existing studies apply deep learning models to make predictions considering only one feature or temporal relationship in load time series. Therefore, to obtain an accurate and reliable prediction result, a hybrid prediction model combining a dual-stage attention mechanism (DA), crisscross grey wolf optimizer (CS-GWO) and bidirectional gated recurrent unit (BiGRU) is proposed in this paper. DA is introduced on the input side of the model to improve the sensitivity of the model to key features and information at key time points simultaneously. CS-GWO is formed by combining the horizontal and vertical crossover operators, to enhance the global search ability and the diversity of the population of GWO. Meanwhile, BiGRU is optimized by CS-GWO to accelerate the convergence of the model. Finally, a collected load dataset, four evaluation metrics and parametric and non-parametric testing manners are used to evaluate the proposed CS-GWO-DA-BiGRU short-term load prediction model. The experimental results show that the RMSE, MAE and SMAPE are reduced respectively by 3.86%, 1.37% and 0.30% of those of the second-best performing CSO-DA-BiGRU model, which demonstrates that the proposed model can better fit the load data and achieve better prediction results.
Record ID
Keywords
crisscross grey wolf optimizer, dual-stage attention mechanism, short-term load prediction
Subject
Suggested Citation
Gong R, Li X. A Short-Term Load Forecasting Model Based on Crisscross Grey Wolf Optimizer and Dual-Stage Attention Mechanism. (2023). LAPSE:2023.31414
Author Affiliations
Gong R: School of Electrical Engineering, Guangxi University, Nanning 530004, China; School of Traffic &Transportation, Nanning University, Nanning 530200, China
Li X: School of Electrical Engineering, Guangxi University, Nanning 530004, China
Li X: School of Electrical Engineering, Guangxi University, Nanning 530004, China
Journal Name
Energies
Volume
16
Issue
6
First Page
2878
Year
2023
Publication Date
2023-03-21
Published Version
ISSN
1996-1073
Version Comments
Original Submission
Other Meta
PII: en16062878, Publication Type: Journal Article
Record Map
Published Article
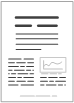
LAPSE:2023.31414
This Record
External Link
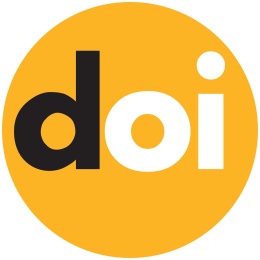
doi:10.3390/en16062878
Publisher Version
Download
Meta
Record Statistics
Record Views
57
Version History
[v1] (Original Submission)
Apr 18, 2023
Verified by curator on
Apr 18, 2023
This Version Number
v1
Citations
Most Recent
This Version
URL Here
https://psecommunity.org/LAPSE:2023.31414
Original Submitter
Auto Uploader for LAPSE
Links to Related Works