LAPSE:2023.31387
Published Article
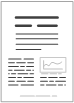
LAPSE:2023.31387
An Artificial Lift Selection Approach Using Machine Learning: A Case Study in Sudan
April 18, 2023
This article presents a machine learning (ML) application to examine artificial lift (AL) selection, using only field production datasets from a Sudanese oil field. Five ML algorithms were used to develop a selection model, and the results demonstrated the ML capabilities in the optimum selection, with accuracy reaching 93%. Moreover, the predicted AL has a better production performance than the actual ones in the field. The research shows the significant production parameters to consider in AL type and size selection. The top six critical factors affecting AL selection are gas, cumulatively produced fluid, wellhead pressure, GOR, produced water, and the implemented EOR. This article contributes significantly to the literature and proposes a new and efficient approach to selecting the optimum AL to maximize oil production and profitability, reducing the analysis time and production losses associated with inconsistency in selection and frequent AL replacement. This study offers a universal model that can be applied to any oil field with different parameters and lifting methods.
Record ID
Keywords
Algorithms, artificial lift, Machine Learning, production data, supervised learning
Subject
Suggested Citation
Mahdi MAA, Amish M, Oluyemi G. An Artificial Lift Selection Approach Using Machine Learning: A Case Study in Sudan. (2023). LAPSE:2023.31387
Author Affiliations
Mahdi MAA: School of Engineering, Robert Gordon University, Garthdee Road, Aberdeen AB10 7GJ, UK [ORCID]
Amish M: School of Engineering, Robert Gordon University, Garthdee Road, Aberdeen AB10 7GJ, UK
Oluyemi G: School of Engineering, Robert Gordon University, Garthdee Road, Aberdeen AB10 7GJ, UK
Amish M: School of Engineering, Robert Gordon University, Garthdee Road, Aberdeen AB10 7GJ, UK
Oluyemi G: School of Engineering, Robert Gordon University, Garthdee Road, Aberdeen AB10 7GJ, UK
Journal Name
Energies
Volume
16
Issue
6
First Page
2853
Year
2023
Publication Date
2023-03-19
Published Version
ISSN
1996-1073
Version Comments
Original Submission
Other Meta
PII: en16062853, Publication Type: Journal Article
Record Map
Published Article
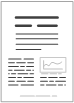
LAPSE:2023.31387
This Record
External Link
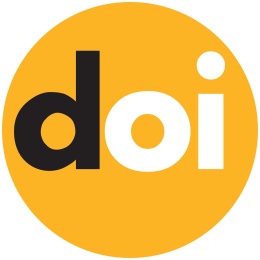
doi:10.3390/en16062853
Publisher Version
Download
Meta
Record Statistics
Record Views
122
Version History
[v1] (Original Submission)
Apr 18, 2023
Verified by curator on
Apr 18, 2023
This Version Number
v1
Citations
Most Recent
This Version
URL Here
https://psecommunity.org/LAPSE:2023.31387
Original Submitter
Auto Uploader for LAPSE
Links to Related Works