LAPSE:2023.31369
Published Article
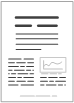
LAPSE:2023.31369
To Charge or to Sell? EV Pack Useful Life Estimation via LSTMs, CNNs, and Autoencoders
April 18, 2023
Electric vehicles (EVs) are spreading fast as they promise to provide better performance and comfort, but above all, to help face climate change. Despite their success, their cost is still a challenge. Lithium-ion batteries are one of the most expensive EV components, and have become the standard for energy storage in various applications. Precisely estimating the remaining useful life (RUL) of battery packs can encourage their reuse and thus help to reduce the cost of EVs and improve sustainability. A correct RUL estimation can be used to quantify the residual market value of the battery pack. The customer can then decide to sell the battery when it still has a value, i.e., before it exceeds the end of life of the target application, so it can still be reused in a second domain without compromising safety and reliability. This paper proposes and compares two deep learning approaches to estimate the RUL of Li-ion batteries: LSTM and autoencoders vs. CNN and autoencoders. The autoencoders are used to extract useful features, while the subsequent network is then used to estimate the RUL. Compared to what has been proposed so far in the literature, we employ measures to ensure the method’s applicability in the actual deployed application. Such measures include (1) avoiding using non-measurable variables as input, (2) employing appropriate datasets with wide variability and different conditions, and (3) predicting the remaining ampere-hours instead of the number of cycles. The results show that the proposed methods can generalize on datasets consisting of numerous batteries with high variance.
Record ID
Keywords
autoencoder, data-driven, deep learning, DL regression, electric vehicle, lithium-ion batteries, LSTM, Machine Learning, remaining useful life, RUL
Subject
Suggested Citation
Bosello M, Falcomer C, Rossi C, Pau G. To Charge or to Sell? EV Pack Useful Life Estimation via LSTMs, CNNs, and Autoencoders. (2023). LAPSE:2023.31369
Author Affiliations
Bosello M: Department of Computer Science and Engineering, University of Bologna, 40126 Bologna, Italy; Autonomous Robotics Research Center, Technology Innovation Institute (TII), Abu Dhabi P.O. Box 9639, United Arab Emirates [ORCID]
Falcomer C: Department of Computer Science and Engineering, University of Bologna, 40126 Bologna, Italy [ORCID]
Rossi C: Department of Electrical, Electronic and Information Engineering, University of Bologna, 40136 Bologna, Italy [ORCID]
Pau G: Department of Computer Science and Engineering, University of Bologna, 40126 Bologna, Italy; Autonomous Robotics Research Center, Technology Innovation Institute (TII), Abu Dhabi P.O. Box 9639, United Arab Emirates; Samueli Computer Science Department, Un [ORCID]
Falcomer C: Department of Computer Science and Engineering, University of Bologna, 40126 Bologna, Italy [ORCID]
Rossi C: Department of Electrical, Electronic and Information Engineering, University of Bologna, 40136 Bologna, Italy [ORCID]
Pau G: Department of Computer Science and Engineering, University of Bologna, 40126 Bologna, Italy; Autonomous Robotics Research Center, Technology Innovation Institute (TII), Abu Dhabi P.O. Box 9639, United Arab Emirates; Samueli Computer Science Department, Un [ORCID]
Journal Name
Energies
Volume
16
Issue
6
First Page
2837
Year
2023
Publication Date
2023-03-18
Published Version
ISSN
1996-1073
Version Comments
Original Submission
Other Meta
PII: en16062837, Publication Type: Journal Article
Record Map
Published Article
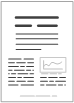
LAPSE:2023.31369
This Record
External Link
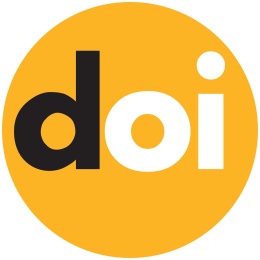
doi:10.3390/en16062837
Publisher Version
Download
Meta
Record Statistics
Record Views
79
Version History
[v1] (Original Submission)
Apr 18, 2023
Verified by curator on
Apr 18, 2023
This Version Number
v1
Citations
Most Recent
This Version
URL Here
https://psecommunity.org/LAPSE:2023.31369
Original Submitter
Auto Uploader for LAPSE
Links to Related Works