LAPSE:2023.31261
Published Article
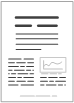
LAPSE:2023.31261
Ultra-Short-Term Prediction Method of Wind Power for Massive Wind Power Clusters Based on Feature Mining of Spatiotemporal Correlation
April 18, 2023
With the centralization of wind power development, power-prediction technology based on wind power clusters has become an important means to reduce the volatility of wind power, so a large-scale power-prediction method of wind power clusters is proposed considering the prediction stability. Firstly, the fluctuating features of wind farms are constructed by acquiring statistical features to further build a divided model of wind power clusters using fuzzy clustering algorithm. Then the spatiotemporal features of the data of wind power are obtained using a spatiotemporal attention network to train the prediction model of wind power clusters in a large scale. Finally, the stability of predictive performance of wind power is analyzed using the comprehensive index evaluation system. The results show that the RMSE of wind power prediction is lower than 0.079 at large-scale wind farms based on the prediction method of wind power proposed in this paper using experience based on the data of 159 wind farms in the Nei Monggol Autonomous Region in China and the extreme error is better than 25% for the total capacity of wind farms, which indicates high stability and accuracy.
Record ID
Keywords
performance evaluation, spatial–temporal attention network, stable operation, ultra-short-term forecasting, wind power clusters in large-scale
Subject
Suggested Citation
Wang B, Wang T, Yang M, Han C, Huang D, Gu D. Ultra-Short-Term Prediction Method of Wind Power for Massive Wind Power Clusters Based on Feature Mining of Spatiotemporal Correlation. (2023). LAPSE:2023.31261
Author Affiliations
Wang B: State Key Laboratory of Operation and Control of Renewable Energy & Storage Systems, China Electric Power Research Institute, Beijing 100192, China
Wang T: Key Laboratory of Modern Power System Simulation and Control & Renewable Energy Technology, Ministry of Education, Northeast Electric Power University, Jilin 132012, China
Yang M: Key Laboratory of Modern Power System Simulation and Control & Renewable Energy Technology, Ministry of Education, Northeast Electric Power University, Jilin 132012, China
Han C: Key Laboratory of Modern Power System Simulation and Control & Renewable Energy Technology, Ministry of Education, Northeast Electric Power University, Jilin 132012, China
Huang D: School of Power Transmission and Distribution, Northeast Electric Power University, Jilin 132012, China
Gu D: School of Automation Engineering, Northeast Electric Power University, Jilin 132012, China
Wang T: Key Laboratory of Modern Power System Simulation and Control & Renewable Energy Technology, Ministry of Education, Northeast Electric Power University, Jilin 132012, China
Yang M: Key Laboratory of Modern Power System Simulation and Control & Renewable Energy Technology, Ministry of Education, Northeast Electric Power University, Jilin 132012, China
Han C: Key Laboratory of Modern Power System Simulation and Control & Renewable Energy Technology, Ministry of Education, Northeast Electric Power University, Jilin 132012, China
Huang D: School of Power Transmission and Distribution, Northeast Electric Power University, Jilin 132012, China
Gu D: School of Automation Engineering, Northeast Electric Power University, Jilin 132012, China
Journal Name
Energies
Volume
16
Issue
6
First Page
2727
Year
2023
Publication Date
2023-03-15
Published Version
ISSN
1996-1073
Version Comments
Original Submission
Other Meta
PII: en16062727, Publication Type: Journal Article
Record Map
Published Article
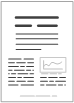
LAPSE:2023.31261
This Record
External Link
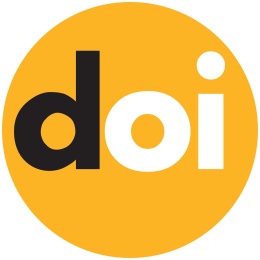
doi:10.3390/en16062727
Publisher Version
Download
Meta
Record Statistics
Record Views
93
Version History
[v1] (Original Submission)
Apr 18, 2023
Verified by curator on
Apr 18, 2023
This Version Number
v1
Citations
Most Recent
This Version
URL Here
https://psecommunity.org/LAPSE:2023.31261
Original Submitter
Auto Uploader for LAPSE
Links to Related Works