LAPSE:2023.30921
Published Article
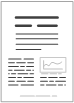
LAPSE:2023.30921
Comparison of Standalone and Hybrid Machine Learning Models for Prediction of Critical Heat Flux in Vertical Tubes
April 17, 2023
Critical heat flux (CHF) is an essential parameter that plays a significant role in ensuring the safety and economic efficiency of nuclear power facilities. It imposes design and operational restrictions on nuclear power plants due to safety concerns. Therefore, accurate prediction of CHF using a hybrid framework can assist researchers in optimizing system performance, mitigating risk of equipment failure, and enhancing safety measures. Despite the existence of numerous prediction methods, there remains a lack of agreement regarding the underlying mechanism that gives rise to CHF. Hence, developing a precise and reliable CHF model is a crucial and challenging task. In this study, we proposed a hybrid model based on an artificial neural network (ANN) to improve the prediction accuracy of CHF. Our model leverages the available knowledge from a lookup table (LUT) and then employs ANN to further reduce the gap between actual and predicted outcomes. To develop and assess the accuracy of our model, we compiled a dataset of around 5877 data points from various sources in the literature. This dataset encompasses a diverse range of operating parameters for two-phase flow in vertical tubes. The results of this study demonstrate that the proposed hybrid model performs better than standalone machine learning models such as ANN, random forest, support vector machine, and data-driven lookup tables, with a relative root-mean-square error (rRMSE) of only 9.3%. We also evaluated the performance of the proposed hybrid model using holdout and cross-validation techniques, which demonstrated its robustness. Moreover, the proposed approach offers valuable insights into the significance of various input parameters in predicting CHF. Our proposed system can be utilized as a real-time monitoring tool for predicting extreme conditions in nuclear reactors, ensuring their safe and efficient operation.
Record ID
Keywords
critical heat flux, flow boiling, lookup table, Machine Learning, multiphase flows
Subject
Suggested Citation
Khalid RZ, Ullah A, Khan A, Khan A, Inayat MH. Comparison of Standalone and Hybrid Machine Learning Models for Prediction of Critical Heat Flux in Vertical Tubes. (2023). LAPSE:2023.30921
Author Affiliations
Khalid RZ: Department of Chemical Engineering, Pakistan Institute of Engineering and Applied Sciences, Nilore, Islamabad 45650, Pakistan; Pattern Recognition Lab (PRLab), Department of Computer and Information Sciences, Pakistan Institute of Engineering and Applied
Ullah A: Department of Chemical Engineering, Pakistan Institute of Engineering and Applied Sciences, Nilore, Islamabad 45650, Pakistan [ORCID]
Khan A: Pattern Recognition Lab (PRLab), Department of Computer and Information Sciences, Pakistan Institute of Engineering and Applied Sciences, Nilore, Islamabad 45650, Pakistan; PIEAS Artificial Intelligence Center (PAIC), Pakistan Institute of Engineering and
Khan A: Sino-French Joint Institute (DCI), Dongguan University of Technology (DGUT), Dongguan 523820, China
Inayat MH: Department of Chemical Engineering, Pakistan Institute of Engineering and Applied Sciences, Nilore, Islamabad 45650, Pakistan
Ullah A: Department of Chemical Engineering, Pakistan Institute of Engineering and Applied Sciences, Nilore, Islamabad 45650, Pakistan [ORCID]
Khan A: Pattern Recognition Lab (PRLab), Department of Computer and Information Sciences, Pakistan Institute of Engineering and Applied Sciences, Nilore, Islamabad 45650, Pakistan; PIEAS Artificial Intelligence Center (PAIC), Pakistan Institute of Engineering and
Khan A: Sino-French Joint Institute (DCI), Dongguan University of Technology (DGUT), Dongguan 523820, China
Inayat MH: Department of Chemical Engineering, Pakistan Institute of Engineering and Applied Sciences, Nilore, Islamabad 45650, Pakistan
Journal Name
Energies
Volume
16
Issue
7
First Page
3182
Year
2023
Publication Date
2023-03-31
Published Version
ISSN
1996-1073
Version Comments
Original Submission
Other Meta
PII: en16073182, Publication Type: Journal Article
Record Map
Published Article
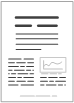
LAPSE:2023.30921
This Record
External Link
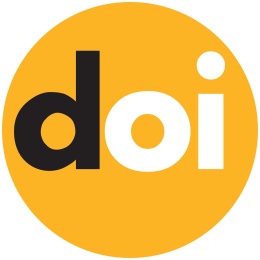
doi:10.3390/en16073182
Publisher Version
Download
Meta
Record Statistics
Record Views
83
Version History
[v1] (Original Submission)
Apr 17, 2023
Verified by curator on
Apr 17, 2023
This Version Number
v1
Citations
Most Recent
This Version
URL Here
https://psecommunity.org/LAPSE:2023.30921
Original Submitter
Auto Uploader for LAPSE
Links to Related Works