LAPSE:2023.30038
Published Article
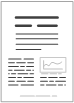
LAPSE:2023.30038
Combination of Thermal Modelling and Machine Learning Approaches for Fault Detection in Wind Turbine Gearboxes
April 14, 2023
This research aims to bring together thermal modelling and machine learning approaches to improve the understanding on the operation and fault detection of a wind turbine gearbox. Recent fault detection research has focused on machine learning, black box approaches. Although it can be successful, it provides no indication of the physical behaviour. In this paper, thermal network modelling was applied to two datasets using SCADA (Supervisory Control and Data Acquisition) temperature data, with the aim of detecting a fault one month before failure. A machine learning approach was used on the same data to compare the results to thermal modelling. The results found that thermal network modelling could successfully detect a fault in many of the turbines examined and was validated by the machine learning approach for one of the datasets. For that same dataset, it was found that combining the thermal model losses and the machine learning approach by using the modelled losses as a feature in the classifier resulted in the engineered feature becoming the most important feature in the classifier. It was also found that the results from thermal modelling had a significantly greater effect on successfully classifying the health of a turbine compared to temperature data. The other dataset gave less conclusive results, suggesting that the location of the fault and the temperature sensors could impact the fault-detection ability.
Record ID
Keywords
condition monitoring, Fault Detection, Machine Learning, thermal modelling, wind energy, wind turbine gearbox
Subject
Suggested Citation
Corley B, Koukoura S, Carroll J, McDonald A. Combination of Thermal Modelling and Machine Learning Approaches for Fault Detection in Wind Turbine Gearboxes. (2023). LAPSE:2023.30038
Author Affiliations
Corley B: Wind Energy & Control Centre, University of Strathclyde, Glasgow G1 2TB, UK [ORCID]
Koukoura S: Wind Energy & Control Centre, University of Strathclyde, Glasgow G1 2TB, UK [ORCID]
Carroll J: Wind Energy & Control Centre, University of Strathclyde, Glasgow G1 2TB, UK
McDonald A: Institute for Energy Systems, University of Edinburgh, Edinburgh EH9 3DW, UK
Koukoura S: Wind Energy & Control Centre, University of Strathclyde, Glasgow G1 2TB, UK [ORCID]
Carroll J: Wind Energy & Control Centre, University of Strathclyde, Glasgow G1 2TB, UK
McDonald A: Institute for Energy Systems, University of Edinburgh, Edinburgh EH9 3DW, UK
Journal Name
Energies
Volume
14
Issue
5
First Page
1375
Year
2021
Publication Date
2021-03-03
Published Version
ISSN
1996-1073
Version Comments
Original Submission
Other Meta
PII: en14051375, Publication Type: Journal Article
Record Map
Published Article
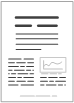
LAPSE:2023.30038
This Record
External Link
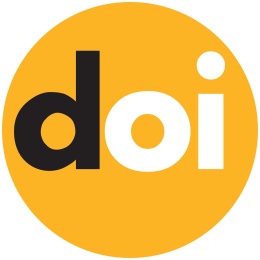
doi:10.3390/en14051375
Publisher Version
Download
Meta
Record Statistics
Record Views
115
Version History
[v1] (Original Submission)
Apr 14, 2023
Verified by curator on
Apr 14, 2023
This Version Number
v1
Citations
Most Recent
This Version
URL Here
https://psecommunity.org/LAPSE:2023.30038
Original Submitter
Auto Uploader for LAPSE
Links to Related Works