LAPSE:2023.29892
Published Article
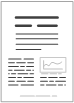
LAPSE:2023.29892
Hybrid and Ensemble Methods of Two Days Ahead Forecasts of Electric Energy Production in a Small Wind Turbine
April 14, 2023
The ability to forecast electricity generation for a small wind turbine is important both on a larger scale where there are many such turbines (because it creates problems for networks managed by distribution system operators) and for prosumers to allow current energy consumption planning. It is also important for owners of small energy systems in order to optimize the use of various energy sources and facilitate energy storage. The research presented here addresses an original, rarely predicted 48 h forecasting horizon for small wind turbines. This topic has been rather underrepresented in research, especially in comparison with forecasts for large wind farms. Wind speed forecasts with a 48 h horizon are also rarely used as input data. We have analyzed the available data to identify potentially useful explanatory variables for forecasting models. Eight sets with increasing data amounts were created to analyze the influence of the types and amounts of data on forecast quality. Hybrid, ensemble and single methods are used for predictions, including machine learning (ML) solutions like long short-term memory (LSTM), multi-layer perceptron (MLP), support vector regression (SVR) and K-nearest neighbours regression (KNNR). Original hybrid methods, developed for research of specific implementations and ensemble methods based on hybrid methods’ decreased errors of energy generation forecasts for small wind turbines in comparison with single methods. The “artificial neural network (ANN) type MLP as an integrator of ensemble based on hybrid methods” ensemble forecasting method incorporates an original combination of predictors. Predictions by this method have the lowest mean absolute error (MAE). In addition, this paper presents an original ensemble forecasting method, called “averaging ensemble based on hybrid methods without extreme forecasts”. Predictions by this method have the lowest root mean square error (RMSE) error among all tested methods. LSTM, a deep neural network, is the best single method, MLP is the second best one, while SVR, KNNR and, especially, linear regression (LR) perform less well. We prove that lagged values of forecasted time series slightly increase the accuracy of predictions. The same applies to seasonal and daily variability markers. Our studies have also demonstrated that using the full set of available input data and the best proposed hybrid and ensemble methods yield the lowest error. The proposed hybrid and ensemble methods are also applicable to other short-time generation forecasting in renewable energy sources (RES), e.g., in photovoltaic (PV) systems or hydropower.
Record ID
Keywords
deep neural network, electric energy production, ensemble methods, hybrid methods, Machine Learning, short-term forecasting, swarm intelligence, wind energy, wind turbine
Suggested Citation
Piotrowski P, Kopyt M, Baczyński D, Robak S, Gulczyński T. Hybrid and Ensemble Methods of Two Days Ahead Forecasts of Electric Energy Production in a Small Wind Turbine. (2023). LAPSE:2023.29892
Author Affiliations
Piotrowski P: Institute of Electrical Power Engineering, Warsaw University of Technology, Koszykowa 75 Street, 00-661 Warszawa, Poland [ORCID]
Kopyt M: Institute of Electrical Power Engineering, Warsaw University of Technology, Koszykowa 75 Street, 00-661 Warszawa, Poland
Baczyński D: Institute of Electrical Power Engineering, Warsaw University of Technology, Koszykowa 75 Street, 00-661 Warszawa, Poland
Robak S: Institute of Electrical Power Engineering, Warsaw University of Technology, Koszykowa 75 Street, 00-661 Warszawa, Poland
Gulczyński T: Globema Sp. z o. o., Wita Stwosza 22 Street, 02-661 Warsaw, Poland
Kopyt M: Institute of Electrical Power Engineering, Warsaw University of Technology, Koszykowa 75 Street, 00-661 Warszawa, Poland
Baczyński D: Institute of Electrical Power Engineering, Warsaw University of Technology, Koszykowa 75 Street, 00-661 Warszawa, Poland
Robak S: Institute of Electrical Power Engineering, Warsaw University of Technology, Koszykowa 75 Street, 00-661 Warszawa, Poland
Gulczyński T: Globema Sp. z o. o., Wita Stwosza 22 Street, 02-661 Warsaw, Poland
Journal Name
Energies
Volume
14
Issue
5
First Page
1225
Year
2021
Publication Date
2021-02-24
Published Version
ISSN
1996-1073
Version Comments
Original Submission
Other Meta
PII: en14051225, Publication Type: Journal Article
Record Map
Published Article
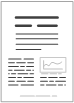
LAPSE:2023.29892
This Record
External Link
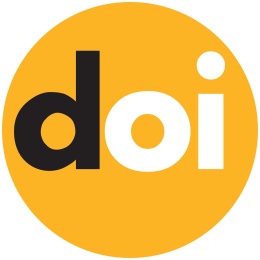
doi:10.3390/en14051225
Publisher Version
Download
Meta
Record Statistics
Record Views
74
Version History
[v1] (Original Submission)
Apr 14, 2023
Verified by curator on
Apr 14, 2023
This Version Number
v1
Citations
Most Recent
This Version
URL Here
https://psecommunity.org/LAPSE:2023.29892
Original Submitter
Auto Uploader for LAPSE
Links to Related Works