LAPSE:2023.29728
Published Article
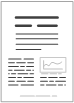
LAPSE:2023.29728
Practical CO2—WAG Field Operational Designs Using Hybrid Numerical-Machine-Learning Approaches
April 13, 2023
Machine-learning technologies have exhibited robust competences in solving many petroleum engineering problems. The accurate predictivity and fast computational speed enable a large volume of time-consuming engineering processes such as history-matching and field development optimization. The Southwest Regional Partnership on Carbon Sequestration (SWP) project desires rigorous history-matching and multi-objective optimization processes, which fits the superiorities of the machine-learning approaches. Although the machine-learning proxy models are trained and validated before imposing to solve practical problems, the error margin would essentially introduce uncertainties to the results. In this paper, a hybrid numerical machine-learning workflow solving various optimization problems is presented. By coupling the expert machine-learning proxies with a global optimizer, the workflow successfully solves the history-matching and CO2 water alternative gas (WAG) design problem with low computational overheads. The history-matching work considers the heterogeneities of multiphase relative characteristics, and the CO2-WAG injection design takes multiple techno-economic objective functions into accounts. This work trained an expert response surface, a support vector machine, and a multi-layer neural network as proxy models to effectively learn the high-dimensional nonlinear data structure. The proposed workflow suggests revisiting the high-fidelity numerical simulator for validation purposes. The experience gained from this work would provide valuable guiding insights to similar CO2 enhanced oil recovery (EOR) projects.
Record ID
Keywords
CO2-WAG, hybrid workflows, Machine Learning, multi-objective optimization, numerical modeling
Subject
Suggested Citation
Sun Q, Ampomah W, You J, Cather M, Balch R. Practical CO2—WAG Field Operational Designs Using Hybrid Numerical-Machine-Learning Approaches. (2023). LAPSE:2023.29728
Author Affiliations
Sun Q: Petroleum Recovery Research Center, New Mexico Institute of Mining and Technology, Socorro, NM 87801, USA; School of Energy Resources, China University of Geoscience, Beijing 100083, China
Ampomah W: Petroleum Recovery Research Center, New Mexico Institute of Mining and Technology, Socorro, NM 87801, USA
You J: Petroleum Recovery Research Center, New Mexico Institute of Mining and Technology, Socorro, NM 87801, USA; School of Petroleum and Natural Gas Engineering, Chongqing University of Science and Technology, Chongqing 401331, China
Cather M: Petroleum Recovery Research Center, New Mexico Institute of Mining and Technology, Socorro, NM 87801, USA
Balch R: Petroleum Recovery Research Center, New Mexico Institute of Mining and Technology, Socorro, NM 87801, USA
Ampomah W: Petroleum Recovery Research Center, New Mexico Institute of Mining and Technology, Socorro, NM 87801, USA
You J: Petroleum Recovery Research Center, New Mexico Institute of Mining and Technology, Socorro, NM 87801, USA; School of Petroleum and Natural Gas Engineering, Chongqing University of Science and Technology, Chongqing 401331, China
Cather M: Petroleum Recovery Research Center, New Mexico Institute of Mining and Technology, Socorro, NM 87801, USA
Balch R: Petroleum Recovery Research Center, New Mexico Institute of Mining and Technology, Socorro, NM 87801, USA
Journal Name
Energies
Volume
14
Issue
4
First Page
1055
Year
2021
Publication Date
2021-02-17
Published Version
ISSN
1996-1073
Version Comments
Original Submission
Other Meta
PII: en14041055, Publication Type: Journal Article
Record Map
Published Article
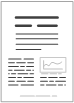
LAPSE:2023.29728
This Record
External Link
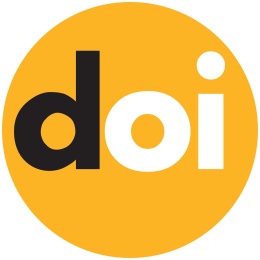
doi:10.3390/en14041055
Publisher Version
Download
Meta
Record Statistics
Record Views
78
Version History
[v1] (Original Submission)
Apr 13, 2023
Verified by curator on
Apr 13, 2023
This Version Number
v1
Citations
Most Recent
This Version
URL Here
https://psecommunity.org/LAPSE:2023.29728
Original Submitter
Auto Uploader for LAPSE
Links to Related Works