LAPSE:2023.29634
Published Article
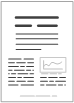
LAPSE:2023.29634
Innovative Methodology to Identify Errors in Electric Energy Measurement Systems in Power Utilities
April 13, 2023
Many electric utilities currently have a low level of smart meter implementation on traditional distribution grids. These utilities commonly have a problem associated with non-technical energy losses (NTLs) to unidentified energy flows consumed, but not billed in power distribution grids. They are usually due to either the electricity theft carried out by their own customers or failures in the utilities’ energy measurement systems. Non-technical energy losses lead to significant economic losses for electric utilities around the world. For instance, in Latin America and the Caribbean countries, NTLs represent around 15% of total energy generated in 2018, varying between 5 and 30% depending on the country because of the strong correlation with social, economic, political, and technical variables. According to this, electric utilities have a strong interest in finding new techniques and methods to mitigate this problem as much as possible. This research presents the results of determining with the precision of the existing data-oriented methods for detecting NTL through a methodology based on data analytics, machine learning, and artificial intelligence (multivariate data, analysis methods, classification, grouping algorithms, i.e., k-means and neural networks). The proposed methodology was implemented using the MATLAB computational tool, demonstrating improvements in the probability to identify the suspected customer’s measurement systems with error in their records that should be revised to reduce the NTLs in the distribution system and using the information from utilities’ databases associated with customer information (customer information system), the distribution grid (geographic information system), and socio-economic data. The proposed methodology was tested and validated in a real situation as a part of a recent Ecuadorian electric project.
Record ID
Keywords
Artificial Intelligence, consumption patterns, data analytics, electrical energy losses, Machine Learning, outlier detection
Subject
Suggested Citation
Toledo-Orozco M, Arias-Marin C, Álvarez-Bel C, Morales-Jadan D, Rodríguez-García J, Bravo-Padilla E. Innovative Methodology to Identify Errors in Electric Energy Measurement Systems in Power Utilities. (2023). LAPSE:2023.29634
Author Affiliations
Toledo-Orozco M: Institute for Energy Engineering, Universitat Politècnica de València, Camino de Vera, 46022 Valencia, Spain; School of Electrical Engineering, Faculty of Engineering, Universidad de Cuenca, Cuenca 010109, Ecuador [ORCID]
Arias-Marin C: Electrical Engineering Career, Universidad Politécnica Salesiana, Sede Cuenca 010103, Ecuador
Álvarez-Bel C: Institute for Energy Engineering, Universitat Politècnica de València, Camino de Vera, 46022 Valencia, Spain
Morales-Jadan D: Electrical Engineering Career, Circular Economy Laboratory-CIITT, Universidad Católica de Cuenca, Sede Cuenca 010107, Ecuador [ORCID]
Rodríguez-García J: Institute for Energy Engineering, Universitat Politècnica de València, Camino de Vera, 46022 Valencia, Spain [ORCID]
Bravo-Padilla E: School of Electrical Engineering, Faculty of Engineering, Universidad de Cuenca, Cuenca 010109, Ecuador
Arias-Marin C: Electrical Engineering Career, Universidad Politécnica Salesiana, Sede Cuenca 010103, Ecuador
Álvarez-Bel C: Institute for Energy Engineering, Universitat Politècnica de València, Camino de Vera, 46022 Valencia, Spain
Morales-Jadan D: Electrical Engineering Career, Circular Economy Laboratory-CIITT, Universidad Católica de Cuenca, Sede Cuenca 010107, Ecuador [ORCID]
Rodríguez-García J: Institute for Energy Engineering, Universitat Politècnica de València, Camino de Vera, 46022 Valencia, Spain [ORCID]
Bravo-Padilla E: School of Electrical Engineering, Faculty of Engineering, Universidad de Cuenca, Cuenca 010109, Ecuador
Journal Name
Energies
Volume
14
Issue
4
First Page
958
Year
2021
Publication Date
2021-02-11
Published Version
ISSN
1996-1073
Version Comments
Original Submission
Other Meta
PII: en14040958, Publication Type: Journal Article
Record Map
Published Article
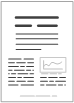
LAPSE:2023.29634
This Record
External Link
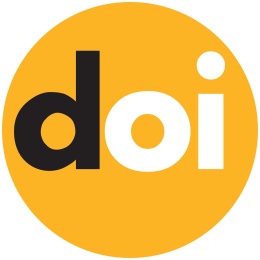
doi:10.3390/en14040958
Publisher Version
Download
Meta
Record Statistics
Record Views
89
Version History
[v1] (Original Submission)
Apr 13, 2023
Verified by curator on
Apr 13, 2023
This Version Number
v1
Citations
Most Recent
This Version
URL Here
https://psecommunity.org/LAPSE:2023.29634
Original Submitter
Auto Uploader for LAPSE
Links to Related Works