LAPSE:2023.29141
Published Article
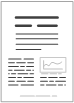
LAPSE:2023.29141
Optimization Techniques for Mining Power Quality Data and Processing Unbalanced Datasets in Machine Learning Applications
April 13, 2023
In recent years, machine learning applications have received increasing interest from power system researchers. The successful performance of these applications is dependent on the availability of extensive and diverse datasets for the training and validation of machine learning frameworks. However, power systems operate at quasi-steady-state conditions for most of the time, and the measurements corresponding to these states provide limited novel knowledge for the development of machine learning applications. In this paper, a data mining approach based on optimization techniques is proposed for filtering root-mean-square (RMS) voltage profiles and identifying unusual measurements within triggerless power quality datasets. Then, datasets with equal representation between event and non-event observations are created so that machine learning algorithms can extract useful insights from the rare but important event observations. The proposed framework is demonstrated and validated with both synthetic signals and field data measurements.
Record ID
Keywords
change detection, data analytics, data mining, filtering, Machine Learning, Optimization, power quality, signal processing, total variation smoothing
Subject
Suggested Citation
Furlani Bastos A, Santoso S. Optimization Techniques for Mining Power Quality Data and Processing Unbalanced Datasets in Machine Learning Applications. (2023). LAPSE:2023.29141
Author Affiliations
Journal Name
Energies
Volume
14
Issue
2
Article Number
en14020463
Year
2021
Publication Date
2021-01-16
Published Version
ISSN
1996-1073
Version Comments
Original Submission
Other Meta
PII: en14020463, Publication Type: Journal Article
Record Map
Published Article
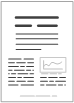
LAPSE:2023.29141
This Record
External Link
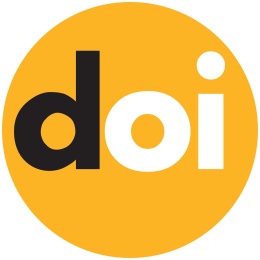
doi:10.3390/en14020463
Publisher Version
Download
Meta
Record Statistics
Record Views
82
Version History
[v1] (Original Submission)
Apr 13, 2023
Verified by curator on
Apr 13, 2023
This Version Number
v1
Citations
Most Recent
This Version
URL Here
https://psecommunity.org/LAPSE:2023.29141
Original Submitter
Auto Uploader for LAPSE
Links to Related Works