LAPSE:2023.29109
Published Article
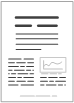
LAPSE:2023.29109
A Novel Application of Ensemble Methods with Data Resampling Techniques for Drill Bit Selection in the Oil and Gas Industry
April 13, 2023
Selection of the most suitable drill bit type is an important task for drillers when planning for new oil and gas wells. With the advancement of intelligent predictive models, the automated selection of drill bit type is possible using earlier drilled offset wells’ data. However, real-field well data samples naturally involve an unequal distribution of data points that results in the formation of a complex imbalance multi-class classification problem during drill bit selection. In this analysis, Ensemble methods, namely Adaboost and Random Forest, have been combined with the data re-sampling techniques to provide a new approach for handling the complex drill bit selection process. Additionally, four popular machine learning techniques namely, K-nearest neighbors, naïve Bayes, multilayer perceptron, and support vector machine, are also evaluated to understand the performance degrading effects of imbalanced drilling data obtained from Norwegian wells. The comparison of results shows that the random forest with bootstrap class weighting technique has given the most impressive performance for bit type selection with testing accuracy ranges from 92% to 99%, and G-mean (0.84−0.97) in critical to normal experimental scenarios. This study provides an approach to automate the drill bit selection process over any field, which will minimize human error, time, and drilling cost.
Record ID
Keywords
drill bits selection, ensemble methods, imbalanced data, petroleum data analytics
Subject
Suggested Citation
Tewari S, Dwivedi UD, Biswas S. A Novel Application of Ensemble Methods with Data Resampling Techniques for Drill Bit Selection in the Oil and Gas Industry. (2023). LAPSE:2023.29109
Author Affiliations
Tewari S: Machine Learning Laboratory, Rajiv Gandhi Institute of Petroleum Technology, Amethi 229304, India [ORCID]
Dwivedi UD: Machine Learning Laboratory, Rajiv Gandhi Institute of Petroleum Technology, Amethi 229304, India [ORCID]
Biswas S: Machine Learning Laboratory, Rajiv Gandhi Institute of Petroleum Technology, Amethi 229304, India [ORCID]
Dwivedi UD: Machine Learning Laboratory, Rajiv Gandhi Institute of Petroleum Technology, Amethi 229304, India [ORCID]
Biswas S: Machine Learning Laboratory, Rajiv Gandhi Institute of Petroleum Technology, Amethi 229304, India [ORCID]
Journal Name
Energies
Volume
14
Issue
2
Article Number
en14020432
Year
2021
Publication Date
2021-01-14
Published Version
ISSN
1996-1073
Version Comments
Original Submission
Other Meta
PII: en14020432, Publication Type: Journal Article
Record Map
Published Article
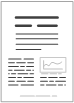
LAPSE:2023.29109
This Record
External Link
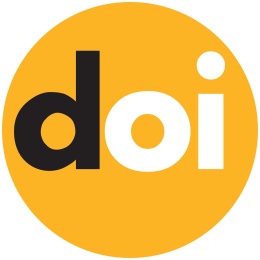
doi:10.3390/en14020432
Publisher Version
Download
Meta
Record Statistics
Record Views
55
Version History
[v1] (Original Submission)
Apr 13, 2023
Verified by curator on
Apr 13, 2023
This Version Number
v1
Citations
Most Recent
This Version
URL Here
https://psecommunity.org/LAPSE:2023.29109
Original Submitter
Auto Uploader for LAPSE
Links to Related Works