LAPSE:2023.28604
Published Article
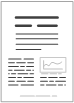
LAPSE:2023.28604
An Integrated Model for Transformer Fault Diagnosis to Improve Sample Classification near Decision Boundary of Support Vector Machine
April 12, 2023
Support vector machine (SVM), which serves as one kind of artificial intelligence technique, has been widely employed in transformer fault diagnosis when involving dissolved gas analysis (DGA). However, when using SVM, it is easy to misclassify samples which are located near the decision boundary, resulting in a decrease in the accuracy of fault diagnosis. Given this issue, this paper proposed a genetic algorithm (GA) optimized probabilistic SVM (GAPSVM) integrated with the fuzzy three-ratio (FTR) method, in which the GAPSVM can judge whether a sample is near the decision boundary according to its output probabilities and diagnose the samples which are not near the decision boundary. Then, FTR is used to diagnose the samples which are near the decision boundary. Combining GAPSVM and FTR, the integrated model can accurately diagnose samples near the decision boundary of SVM. In addition, to avoid redundant and erroneous features, this paper also used GA to select the optimal DGA features. The diagnostic accuracy of the proposed GAPSVM integrated with the FTR fault diagnosis method reached 86.80% after 10 repeated calculations using 118 groups of IEC technical committee (TC) 10 samples. Moreover, the robustness is also proven through 30 groups of DGA samples from the State Grid Co. of China and 15 practical cases with missing values.
Record ID
Keywords
dissolved gas analysis feature, expert experience, fault diagnosis, Genetic Algorithm, power transformer, probabilistic support vector machine
Subject
Suggested Citation
Zhang Y, Wang Y, Fan X, Zhang W, Zhuo R, Hao J, Shi Z. An Integrated Model for Transformer Fault Diagnosis to Improve Sample Classification near Decision Boundary of Support Vector Machine. (2023). LAPSE:2023.28604
Author Affiliations
Zhang Y: Guangxi Key Laboratory of Power System Optimization and Energy Technology, Guangxi University, Nanning 530004, China [ORCID]
Wang Y: Guangxi Key Laboratory of Power System Optimization and Energy Technology, Guangxi University, Nanning 530004, China
Fan X: Guangxi Key Laboratory of Power System Optimization and Energy Technology, Guangxi University, Nanning 530004, China
Zhang W: Guangxi Power Grid Co., Ltd. Electric Power Research Institute, Nanning 530000, China
Zhuo R: Electric Power Research Institute of China Southern Power Grid Company Limited, Guangzhou 510080, China
Hao J: School of Electrical Engineering, Chongqing University, Chongqing 400030, China [ORCID]
Shi Z: Guangxi Key Laboratory of Power System Optimization and Energy Technology, Guangxi University, Nanning 530004, China
Wang Y: Guangxi Key Laboratory of Power System Optimization and Energy Technology, Guangxi University, Nanning 530004, China
Fan X: Guangxi Key Laboratory of Power System Optimization and Energy Technology, Guangxi University, Nanning 530004, China
Zhang W: Guangxi Power Grid Co., Ltd. Electric Power Research Institute, Nanning 530000, China
Zhuo R: Electric Power Research Institute of China Southern Power Grid Company Limited, Guangzhou 510080, China
Hao J: School of Electrical Engineering, Chongqing University, Chongqing 400030, China [ORCID]
Shi Z: Guangxi Key Laboratory of Power System Optimization and Energy Technology, Guangxi University, Nanning 530004, China
Journal Name
Energies
Volume
13
Issue
24
Article Number
E6678
Year
2020
Publication Date
2020-12-17
Published Version
ISSN
1996-1073
Version Comments
Original Submission
Other Meta
PII: en13246678, Publication Type: Journal Article
Record Map
Published Article
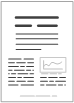
LAPSE:2023.28604
This Record
External Link
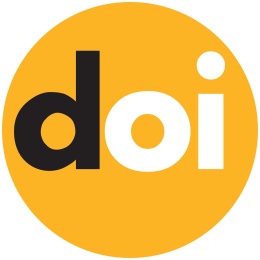
doi:10.3390/en13246678
Publisher Version
Download
Meta
Record Statistics
Record Views
76
Version History
[v1] (Original Submission)
Apr 12, 2023
Verified by curator on
Apr 12, 2023
This Version Number
v1
Citations
Most Recent
This Version
URL Here
https://psecommunity.org/LAPSE:2023.28604
Original Submitter
Auto Uploader for LAPSE
Links to Related Works