LAPSE:2023.28135
Published Article
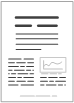
LAPSE:2023.28135
Are Neural Networks the Right Tool for Process Modeling and Control of Batch and Batch-like Processes?
April 11, 2023
The prevalence of batch and batch-like operations, in conjunction with the continued resurgence of artificial intelligence techniques for clustering and classification applications, has increasingly motivated the exploration of the applicability of deep learning for modeling and feedback control of batch and batch-like processes. To this end, the present study seeks to evaluate the viability of artificial intelligence in general, and neural networks in particular, toward process modeling and control via a case study. Nonlinear autoregressive with exogeneous input (NARX) networks are evaluated in comparison with subspace models within the framework of model-based control. A batch polymethyl methacrylate (PMMA) polymerization process is chosen as a simulation test-bed. Subspace-based state-space models and NARX networks identified for the process are first compared for their predictive power. The identified models are then implemented in model predictive control (MPC) to compare the control performance for both modeling approaches. The comparative analysis reveals that the state-space models performed better than NARX networks in predictive power and control performance. Moreover, the NARX networks were found to be less versatile than state-space models in adapting to new process operation. The results of the study indicate that further research is needed before neural networks may become readily applicable for the feedback control of batch processes.
Record ID
Keywords
data-driven model identification, neural networks, subspace identification
Subject
Suggested Citation
Rashid M, Mhaskar P. Are Neural Networks the Right Tool for Process Modeling and Control of Batch and Batch-like Processes?. (2023). LAPSE:2023.28135
Author Affiliations
Rashid M: Department of Chemical Engineering, McMaster University, Hamilton, ON L8S 4L8, Canada
Mhaskar P: Department of Chemical Engineering, McMaster University, Hamilton, ON L8S 4L8, Canada [ORCID]
Mhaskar P: Department of Chemical Engineering, McMaster University, Hamilton, ON L8S 4L8, Canada [ORCID]
Journal Name
Processes
Volume
11
Issue
3
First Page
686
Year
2023
Publication Date
2023-02-24
Published Version
ISSN
2227-9717
Version Comments
Original Submission
Other Meta
PII: pr11030686, Publication Type: Journal Article
Record Map
Published Article
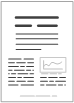
LAPSE:2023.28135
This Record
External Link
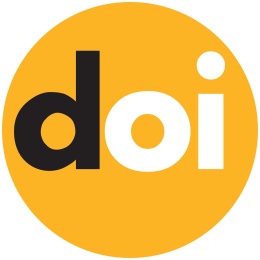
doi:10.3390/pr11030686
Publisher Version
Download
Meta
Record Statistics
Record Views
73
Version History
[v1] (Original Submission)
Apr 11, 2023
Verified by curator on
Apr 11, 2023
This Version Number
v1
Citations
Most Recent
This Version
URL Here
https://psecommunity.org/LAPSE:2023.28135
Original Submitter
Auto Uploader for LAPSE
Links to Related Works