LAPSE:2023.25925
Published Article
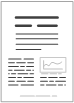
LAPSE:2023.25925
Data Augmentation for Electricity Theft Detection Using Conditional Variational Auto-Encoder
March 31, 2023
Due to the strong concealment of electricity theft and the limitation of inspection resources, the number of power theft samples mastered by the power department is insufficient, which limits the accuracy of power theft detection. Therefore, a data augmentation method for electricity theft detection based on the conditional variational auto-encoder (CVAE) is proposed. Firstly, the stealing power curves are mapped into low dimensional latent variables by using the encoder composed of convolutional layers, and the new stealing power curves are reconstructed by the decoder composed of deconvolutional layers. Then, five typical attack models are proposed, and the convolutional neural network is constructed as a classifier according to the data characteristics of stealing power curves. Finally, the effectiveness and adaptability of the proposed method is verified by a smart meters’ data set from London. The simulation results show that the CVAE can take into account the shapes and distribution characteristics of samples at the same time, and the generated stealing power curves have the best effect on the performance improvement of the classifier than the traditional augmentation methods such as the random oversampling method, synthetic minority over-sampling technique, and conditional generative adversarial network. Moreover, it is suitable for different classifiers.
Record ID
Keywords
conditional variational auto-encoder, convolutional neural network, data augmentation, deep learning, power theft detection
Suggested Citation
Gong X, Tang B, Zhu R, Liao W, Song L. Data Augmentation for Electricity Theft Detection Using Conditional Variational Auto-Encoder. (2023). LAPSE:2023.25925
Author Affiliations
Gong X: Electric Engineering College, Tibet Agriculture and Animal Husbandry University, Nyingchi 860000, China
Tang B: Electric Engineering College, Tibet Agriculture and Animal Husbandry University, Nyingchi 860000, China
Zhu R: Electric Engineering College, Tibet Agriculture and Animal Husbandry University, Nyingchi 860000, China
Liao W: Key Laboratory of Smart Grid of Ministry of Education, Tianjin University, Tianjin 300072, China [ORCID]
Song L: Maintenance Branch of State Grid Jibei Electric Power Co., Ltd., Beijing 102488, China
Tang B: Electric Engineering College, Tibet Agriculture and Animal Husbandry University, Nyingchi 860000, China
Zhu R: Electric Engineering College, Tibet Agriculture and Animal Husbandry University, Nyingchi 860000, China
Liao W: Key Laboratory of Smart Grid of Ministry of Education, Tianjin University, Tianjin 300072, China [ORCID]
Song L: Maintenance Branch of State Grid Jibei Electric Power Co., Ltd., Beijing 102488, China
Journal Name
Energies
Volume
13
Issue
17
Article Number
E4291
Year
2020
Publication Date
2020-08-19
Published Version
ISSN
1996-1073
Version Comments
Original Submission
Other Meta
PII: en13174291, Publication Type: Journal Article
Record Map
Published Article
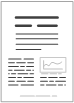
LAPSE:2023.25925
This Record
External Link
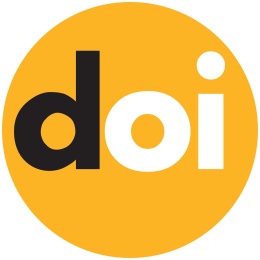
doi:10.3390/en13174291
Publisher Version
Download
Meta
Record Statistics
Record Views
77
Version History
[v1] (Original Submission)
Mar 31, 2023
Verified by curator on
Mar 31, 2023
This Version Number
v1
Citations
Most Recent
This Version
URL Here
https://psecommunity.org/LAPSE:2023.25925
Original Submitter
Auto Uploader for LAPSE
Links to Related Works