LAPSE:2023.25548
Published Article
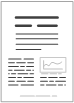
LAPSE:2023.25548
Integrating Machine/Deep Learning Methods and Filtering Techniques for Reliable Mineral Phase Segmentation of 3D X-ray Computed Tomography Images
March 28, 2023
X-ray CT imaging provides a 3D view of a sample and is a powerful tool for investigating the internal features of porous rock. Reliable phase segmentation in these images is highly necessary but, like any other digital rock imaging technique, is time-consuming, labor-intensive, and subjective. Combining 3D X-ray CT imaging with machine learning methods that can simultaneously consider several extracted features in addition to color attenuation, is a promising and powerful method for reliable phase segmentation. Machine learning-based phase segmentation of X-ray CT images enables faster data collection and interpretation than traditional methods. This study investigates the performance of several filtering techniques with three machine learning methods and a deep learning method to assess the potential for reliable feature extraction and pixel-level phase segmentation of X-ray CT images. Features were first extracted from images using well-known filters and from the second convolutional layer of the pre-trained VGG16 architecture. Then, K-means clustering, Random Forest, and Feed Forward Artificial Neural Network methods, as well as the modified U-Net model, were applied to the extracted input features. The models’ performances were then compared and contrasted to determine the influence of the machine learning method and input features on reliable phase segmentation. The results showed considering more dimensionality has promising results and all classification algorithms result in high accuracy ranging from 0.87 to 0.94. Feature-based Random Forest demonstrated the best performance among the machine learning models, with an accuracy of 0.88 for Mancos and 0.94 for Marcellus. The U-Net model with the linear combination of focal and dice loss also performed well with an accuracy of 0.91 and 0.93 for Mancos and Marcellus, respectively. In general, considering more features provided promising and reliable segmentation results that are valuable for analyzing the composition of dense samples, such as shales, which are significant unconventional reservoirs in oil recovery.
Record ID
Keywords
3D imaging of shale samples, 3D X-ray computed tomography, feed-forward neural network, Mancos, Marcellus, random forest, U-Net convolutional neural network
Suggested Citation
Asadi P, Beckingham LE. Integrating Machine/Deep Learning Methods and Filtering Techniques for Reliable Mineral Phase Segmentation of 3D X-ray Computed Tomography Images. (2023). LAPSE:2023.25548
Author Affiliations
Asadi P: Department of Civil & Environmental Engineering, Auburn University, Auburn, AL 36830, USA
Beckingham LE: Department of Civil & Environmental Engineering, Auburn University, Auburn, AL 36830, USA [ORCID]
Beckingham LE: Department of Civil & Environmental Engineering, Auburn University, Auburn, AL 36830, USA [ORCID]
Journal Name
Energies
Volume
14
Issue
15
First Page
4595
Year
2021
Publication Date
2021-07-29
Published Version
ISSN
1996-1073
Version Comments
Original Submission
Other Meta
PII: en14154595, Publication Type: Journal Article
Record Map
Published Article
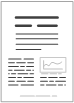
LAPSE:2023.25548
This Record
External Link
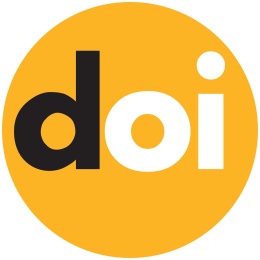
doi:10.3390/en14154595
Publisher Version
Download
Meta
Record Statistics
Record Views
47
Version History
[v1] (Original Submission)
Mar 28, 2023
Verified by curator on
Mar 28, 2023
This Version Number
v1
Citations
Most Recent
This Version
URL Here
https://psecommunity.org/LAPSE:2023.25548
Original Submitter
Auto Uploader for LAPSE
Links to Related Works