LAPSE:2023.23374v1
Published Article
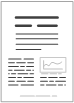
LAPSE:2023.23374v1
Hybrid Machine Learning Models for Classifying Power Quality Disturbances: A Comparative Study
March 27, 2023
The economic impact associated with power quality (PQ) problems in electrical systems is increasing, so PQ improvement research becomes a key task. In this paper, a Stockwell transform (ST)-based hybrid machine learning approach was used for the recognition and classification of power quality disturbances (PQDs). The ST of the PQDs was used to extract significant waveform features which constitute the input vectors for different machine learning approaches, including the K-nearest neighbors’ algorithm (K-NN), decision tree (DT), and support vector machine (SVM) used for classifying the PQDs. The procedure was optimized by using the genetic algorithm (GA) and the competitive swarm optimization algorithm (CSO). To test the proposed methodology, synthetic PQD waveforms were generated. Typical single disturbances for the voltage signal, as well as complex disturbances resulting from possible combinations of them, were considered. Furthermore, different levels of white Gaussian noise were added to the PQD waveforms while maintaining the desired accuracy level of the proposed classification methods. Finally, all the hybrid classification proposals were evaluated and the best one was compared with some others present in the literature. The proposed ST-based CSO-SVM method provides good results in terms of classification accuracy and noise immunity.
Record ID
Keywords
classification, decision tree, feature selection, Genetic Algorithm, K-NN algorithm, power quality disturbances, S-transform, support vector machine, swarm optimization
Subject
Suggested Citation
Bravo-Rodríguez JC, Torres FJ, Borrás MD. Hybrid Machine Learning Models for Classifying Power Quality Disturbances: A Comparative Study. (2023). LAPSE:2023.23374v1
Author Affiliations
Bravo-Rodríguez JC: Escuela Politécnica Superior, Universidad de Sevilla, c/ Virgen de África 9, 41011 Sevilla, Spain [ORCID]
Torres FJ: Escuela Politécnica Superior, Universidad de Sevilla, c/ Virgen de África 9, 41011 Sevilla, Spain
Borrás MD: Escuela Politécnica Superior, Universidad de Sevilla, c/ Virgen de África 9, 41011 Sevilla, Spain
Torres FJ: Escuela Politécnica Superior, Universidad de Sevilla, c/ Virgen de África 9, 41011 Sevilla, Spain
Borrás MD: Escuela Politécnica Superior, Universidad de Sevilla, c/ Virgen de África 9, 41011 Sevilla, Spain
Journal Name
Energies
Volume
13
Issue
11
Article Number
E2761
Year
2020
Publication Date
2020-06-01
Published Version
ISSN
1996-1073
Version Comments
Original Submission
Other Meta
PII: en13112761, Publication Type: Journal Article
Record Map
Published Article
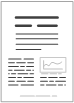
LAPSE:2023.23374v1
This Record
External Link
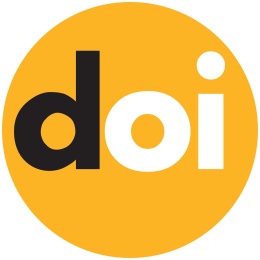
doi:10.3390/en13112761
Publisher Version
Download
Meta
Record Statistics
Record Views
93
Version History
[v1] (Original Submission)
Mar 27, 2023
Verified by curator on
Mar 27, 2023
This Version Number
v1
Citations
Most Recent
This Version
URL Here
https://psecommunity.org/LAPSE:2023.23374v1
Original Submitter
Auto Uploader for LAPSE
Links to Related Works