LAPSE:2023.22532
Published Article
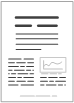
LAPSE:2023.22532
Noise Reduction Power Stealing Detection Model Based on Self-Balanced Data Set
March 24, 2023
In recent years, various types of power theft incidents have occurred frequently, and the training of the power-stealing detection model is susceptible to the influence of the imbalanced data set and the data noise, which leads to errors in power-stealing detection. Therefore, a power-stealing detection model is proposed, which is based on Improved Conditional Generation Adversarial Network (CWGAN), Stacked Convolution Noise Reduction Autoencoder (SCDAE) and Lightweight Gradient Boosting Decision Machine (LightGBM). The model performs Generation- Adversarial operations on the original unbalanced power consumption data to achieve the balance of electricity data, and avoids the interference of the imbalanced data set on classifier training. In addition, the convolution method is used to stack the noise reduction auto-encoder to achieve dimension reduction of power consumption data, extract data features and reduce the impact of random noise. Finally, LightGBM is used for power theft detection. The experiments show that CWGAN can effectively balance the distribution of power consumption data. Comparing the detection indicators of the power-stealing model with various advanced power-stealing models on the same data set, it is finally proved that the proposed model is superior to other models in the detection of power stealing.
Record ID
Keywords
conditional generation network, data set imbalance, LightGBM, power theft detection, stacked convolution noise reduction encoder
Subject
Suggested Citation
Liu H, Li Z, Li Y. Noise Reduction Power Stealing Detection Model Based on Self-Balanced Data Set. (2023). LAPSE:2023.22532
Author Affiliations
Liu H: School of Control and Computer, North China Electric Power University, Beijing 102206, China
Li Z: School of Control and Computer, North China Electric Power University, Beijing 102206, China
Li Y: School of Control and Computer, North China Electric Power University, Beijing 102206, China
Li Z: School of Control and Computer, North China Electric Power University, Beijing 102206, China
Li Y: School of Control and Computer, North China Electric Power University, Beijing 102206, China
Journal Name
Energies
Volume
13
Issue
7
Article Number
E1763
Year
2020
Publication Date
2020-04-07
Published Version
ISSN
1996-1073
Version Comments
Original Submission
Other Meta
PII: en13071763, Publication Type: Journal Article
Record Map
Published Article
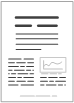
LAPSE:2023.22532
This Record
External Link
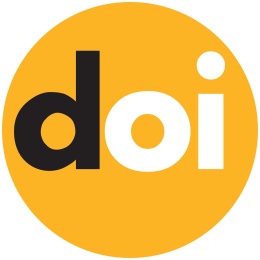
doi:10.3390/en13071763
Publisher Version
Download
Meta
Record Statistics
Record Views
71
Version History
[v1] (Original Submission)
Mar 24, 2023
Verified by curator on
Mar 24, 2023
This Version Number
v1
Citations
Most Recent
This Version
URL Here
https://psecommunity.org/LAPSE:2023.22532
Original Submitter
Auto Uploader for LAPSE
Links to Related Works