LAPSE:2023.21540
Published Article
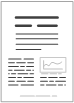
LAPSE:2023.21540
A RBFNN & GACMOO-Based Working State Optimization Control Study on Heavy-Duty Diesel Engine Working in Plateau Environment
March 22, 2023
In order to solve issues concerning performance induction and in-cylinder heat accumulation of a certain heavy-duty diesel engine in a plateau environment, working state parameters and performance indexes of diesel engine are calculated and optimized using the method of artificial neural network and genetic algorithm cycle multi-objective optimization. First, with an established diesel engine simulation model and an orthogonal experimental method, the influence rule of five performance indexes affected by five working state parameters are calculated and analyzed. Results indicate the first four of five working state parameters have a more prominent influence on those five performance indexes. Subsequently, further calculation generates correspondences among four working state parameters and five performance indexes with the method of radial basis function neural network. The predicted value of the trained neural network matches well with the original one. The approach can fulfill serialization of discrete working state parameters and performance indexes to facilitate subsequent analysis and optimization. Next, we came up with a new algorithm named RBFNN & GACMOO, which can calculate the optimal working state parameters and the corresponding performance indexes of the diesel engine working at 3700 m altitude. At last, the bench test of the diesel engine in a plateau environment is employed to verify accuracy of the optimized results and the effectiveness of the algorithm. The research first combined the method of artificial neural network and genetic algorithm to specify the optimal working state parameters of the diesel engine at high altitudes by focusing on engine power, torque and heat dissipation, which is of great significance for improving both performance and working reliability of heavy-duty diesel engine working in plateau environment.
Record ID
Keywords
diesel engine, Genetic Algorithm, multi-objective optimization, plateau, radial basis function neural network
Subject
Suggested Citation
Dong Y, Liu J, Liu Y, Qiao X, Zhang X, Jin Y, Zhang S, Wang T, Kang Q. A RBFNN & GACMOO-Based Working State Optimization Control Study on Heavy-Duty Diesel Engine Working in Plateau Environment. (2023). LAPSE:2023.21540
Author Affiliations
Dong Y: Vehicle Engineering Department, Army Academy of Armored Forces, Beijing 100072, China
Liu J: Vehicle Engineering Department, Army Academy of Armored Forces, Beijing 100072, China
Liu Y: Vehicle Engineering Department, Army Academy of Armored Forces, Beijing 100072, China
Qiao X: Vehicle Engineering Department, Army Academy of Armored Forces, Beijing 100072, China
Zhang X: Vehicle Engineering Department, Army Academy of Armored Forces, Beijing 100072, China
Jin Y: Vehicle Engineering Department, Army Academy of Armored Forces, Beijing 100072, China
Zhang S: Vehicle Engineering Department, Army Academy of Armored Forces, Beijing 100072, China
Wang T: Vehicle Engineering Department, Army Academy of Armored Forces, Beijing 100072, China
Kang Q: China Satellite Maritime Tracking and Controlling Department, Wuxi 214431, China
Liu J: Vehicle Engineering Department, Army Academy of Armored Forces, Beijing 100072, China
Liu Y: Vehicle Engineering Department, Army Academy of Armored Forces, Beijing 100072, China
Qiao X: Vehicle Engineering Department, Army Academy of Armored Forces, Beijing 100072, China
Zhang X: Vehicle Engineering Department, Army Academy of Armored Forces, Beijing 100072, China
Jin Y: Vehicle Engineering Department, Army Academy of Armored Forces, Beijing 100072, China
Zhang S: Vehicle Engineering Department, Army Academy of Armored Forces, Beijing 100072, China
Wang T: Vehicle Engineering Department, Army Academy of Armored Forces, Beijing 100072, China
Kang Q: China Satellite Maritime Tracking and Controlling Department, Wuxi 214431, China
Journal Name
Energies
Volume
13
Issue
1
Article Number
E279
Year
2020
Publication Date
2020-01-06
Published Version
ISSN
1996-1073
Version Comments
Original Submission
Other Meta
PII: en13010279, Publication Type: Journal Article
Record Map
Published Article
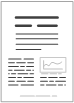
LAPSE:2023.21540
This Record
External Link
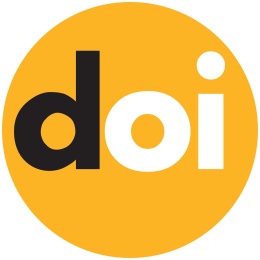
doi:10.3390/en13010279
Publisher Version
Download
Meta
Record Statistics
Record Views
95
Version History
[v1] (Original Submission)
Mar 22, 2023
Verified by curator on
Mar 22, 2023
This Version Number
v1
Citations
Most Recent
This Version
URL Here
https://psecommunity.org/LAPSE:2023.21540
Original Submitter
Auto Uploader for LAPSE
Links to Related Works