LAPSE:2023.18901
Published Article
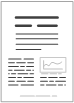
LAPSE:2023.18901
A Meta-Modeling Power Consumption Forecasting Approach Combining Client Similarity and Causality
March 9, 2023
Power forecasting models offer valuable insights on the electricity consumption patterns of clients, enabling the development of advanced strategies and applications aimed at energy saving, increased energy efficiency, and smart energy pricing. The data collection process for client consumption models is not always ideal and the resulting datasets often lead to compromises in the implementation of forecasting models, as well as suboptimal performance, due to several challenges. Therefore, combinations of elements that highlight relationships between clients need to be investigated in order to achieve more accurate consumption predictions. In this study, we exploited the combined effects of client similarity and causality, and developed a power consumption forecasting model that utilizes ensembles of long short-term memory (LSTM) networks. Our novel approach enables the derivation of different representations of the predicted consumption based on feature sets influenced by similarity and causality metrics. The resulting representations were used to train a meta-model, based on a multi-layer perceptron (MLP), in order to combine the results of the LSTM ensembles optimally. This combinatorial approach achieved better overall performance and yielded lower mean absolute percentage error when compared to the standalone LSTM ensembles that do not include similarity and causality. Additional experiments indicated that the combination of similarity and causality resulted in more performant models when compared to implementations utilizing only one element on the same model structure.
Record ID
Keywords
Artificial Intelligence, data analysis, Energy, ensemble neural networks, feature engineering, Machine Learning, meta-modeling, neural networks, power forecasting
Suggested Citation
Kontogiannis D, Bargiotas D, Daskalopulu A, Tsoukalas LH. A Meta-Modeling Power Consumption Forecasting Approach Combining Client Similarity and Causality. (2023). LAPSE:2023.18901
Author Affiliations
Kontogiannis D: Department of Electrical and Computer Engineering, School of Engineering, University of Thessaly, 38221 Volos, Greece
Bargiotas D: Department of Electrical and Computer Engineering, School of Engineering, University of Thessaly, 38221 Volos, Greece
Daskalopulu A: Department of Electrical and Computer Engineering, School of Engineering, University of Thessaly, 38221 Volos, Greece [ORCID]
Tsoukalas LH: AI Systems Lab, School of Nuclear Engineering, Purdue University, West Lafayette, IN 47907, USA
Bargiotas D: Department of Electrical and Computer Engineering, School of Engineering, University of Thessaly, 38221 Volos, Greece
Daskalopulu A: Department of Electrical and Computer Engineering, School of Engineering, University of Thessaly, 38221 Volos, Greece [ORCID]
Tsoukalas LH: AI Systems Lab, School of Nuclear Engineering, Purdue University, West Lafayette, IN 47907, USA
Journal Name
Energies
Volume
14
Issue
19
First Page
6088
Year
2021
Publication Date
2021-09-24
Published Version
ISSN
1996-1073
Version Comments
Original Submission
Other Meta
PII: en14196088, Publication Type: Journal Article
Record Map
Published Article
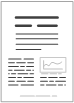
LAPSE:2023.18901
This Record
External Link
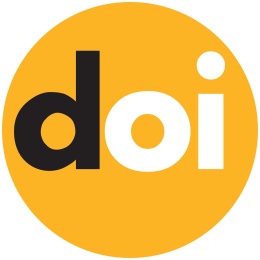
doi:10.3390/en14196088
Publisher Version
Download
Meta
Record Statistics
Record Views
92
Version History
[v1] (Original Submission)
Mar 9, 2023
Verified by curator on
Mar 9, 2023
This Version Number
v1
Citations
Most Recent
This Version
URL Here
https://psecommunity.org/LAPSE:2023.18901
Original Submitter
Auto Uploader for LAPSE
Links to Related Works