LAPSE:2023.17785
Published Article
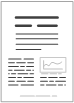
LAPSE:2023.17785
Physics-Based Deep Learning for Flow Problems
March 6, 2023
It is the tradition for the fluid community to study fluid dynamics problems via numerical simulations such as finite-element, finite-difference and finite-volume methods. These approaches use various mesh techniques to discretize a complicated geometry and eventually convert governing equations into finite-dimensional algebraic systems. To date, many attempts have been made by exploiting machine learning to solve flow problems. However, conventional data-driven machine learning algorithms require heavy inputs of large labeled data, which is computationally expensive for complex and multi-physics problems. In this paper, we proposed a data-free, physics-driven deep learning approach to solve various low-speed flow problems and demonstrated its robustness in generating reliable solutions. Instead of feeding neural networks large labeled data, we exploited the known physical laws and incorporated this physics into a neural network to relax the strict requirement of big data and improve prediction accuracy. The employed physics-informed neural networks (PINNs) provide a feasible and cheap alternative to approximate the solution of differential equations with specified initial and boundary conditions. Approximate solutions of physical equations can be obtained via the minimization of the customized objective function, which consists of residuals satisfying differential operators, the initial/boundary conditions as well as the mean-squared errors between predictions and target values. This new approach is data efficient and can greatly lower the computational cost for large and complex geometries. The capacity and generality of the proposed method have been assessed by solving various flow and transport problems, including the flow past cylinder, linear Poisson, heat conduction and the Taylor−Green vortex problem.
Record ID
Keywords
automatic differentiation, deep learning, partial differential equation, physics-informed neural networks, Surrogate Model
Suggested Citation
Sun Y, Sun Q, Qin K. Physics-Based Deep Learning for Flow Problems. (2023). LAPSE:2023.17785
Author Affiliations
Sun Y: Department of Engineering, University of Cambridge, Cambridge CB2 1TN, UK
Sun Q: Investment Promotion and Enterprise Service Center of Yantian District, Shenzhen 518000, China
Qin K: School of Marine Science and Technology, Northwestern Polytechnical University, Xi’an 710060, China
Sun Q: Investment Promotion and Enterprise Service Center of Yantian District, Shenzhen 518000, China
Qin K: School of Marine Science and Technology, Northwestern Polytechnical University, Xi’an 710060, China
Journal Name
Energies
Volume
14
Issue
22
First Page
7760
Year
2021
Publication Date
2021-11-19
Published Version
ISSN
1996-1073
Version Comments
Original Submission
Other Meta
PII: en14227760, Publication Type: Journal Article
Record Map
Published Article
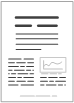
LAPSE:2023.17785
This Record
External Link
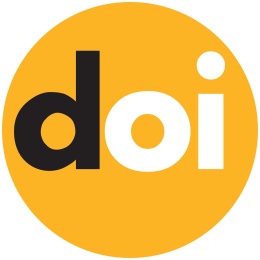
doi:10.3390/en14227760
Publisher Version
Download
Meta
Record Statistics
Record Views
97
Version History
[v1] (Original Submission)
Mar 6, 2023
Verified by curator on
Mar 6, 2023
This Version Number
v1
Citations
Most Recent
This Version
URL Here
https://psecommunity.org/LAPSE:2023.17785
Original Submitter
Auto Uploader for LAPSE
Links to Related Works