LAPSE:2023.1657
Published Article
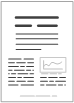
LAPSE:2023.1657
A Novel Multi-Sensor Data-Driven Approach to Source Term Estimation of Hazardous Gas Leakages in the Chemical Industry
February 21, 2023
Source term estimation (STE) is crucial for understanding and addressing hazardous gas leakages in the chemical industry. Most existing methods basically use an atmospheric transport and dispersion (ATD) model to predict the concentrations of hazardous gas leakages from different possible sources, compare the predicted results with multi-sensor data, and use the deviations to search and derive information on the real sources of leakages. Although performing well in principle, complicated computations and the associated computer time often make these methods difficult to apply in real time. Recently, many machine learning methods have also been proposed for the purpose of STE. The idea is to build offline a machine-learning-based STE model using data generated with a high-fidelity ATD model and then apply the machine learning model to multi-sensor data to perform STE in real time. The key to the success of a machine-learning-based STE is that the machine-learning-based STE model has to cover all possible scenarios of concern, which is often difficult in practice because of unpredictable environmental conditions and the inherent robust problems with many supervised machine learning methods. In order to address challenges with the existing STE methods, in the present study, a novel multi-sensor data-driven approach to STE of hazardous gas leakages is proposed. The basic idea is to establish a multi-sensor data-driven STE model from historical multi-sensor observations that cover the situations known as the independent hazardous-gas-leakage scenarios (IHGLSs) in a chemical industry park of concern. Then the established STE model is applied to online process multi-sensor data and perform STE for the chemical industry park in real time. The new approach is based on a rigorous analysis of the relationship between multi-sensor data and sources of hazardous gas leakages and derived using advanced data science, including unsupervised multi-sensor data clustering and analysis. As an example of demonstration, the proposed approach is applied to perform STE for hazardous gas-leakage scenarios wherein a Gaussian plume model can be used to describe the atmospheric transport and dispersion. Because of no need of ATD-model-based online optimization and supervised machine learning, the new approach can potentially overcome many problems with existing methods and enable STE to be literally applied in engineering practice.
Record ID
Keywords
independent hazardous-gas-leakage scenarios (IHGLSs), multi-sensor data-driven, real-time experimental observations and implementation, source term estimation, unsupervised multi-sensor data clustering and analysis
Subject
Suggested Citation
Lang Z, Wang B, Wang Y, Cao C, Peng X, Du W, Qian F. A Novel Multi-Sensor Data-Driven Approach to Source Term Estimation of Hazardous Gas Leakages in the Chemical Industry. (2023). LAPSE:2023.1657
Author Affiliations
Lang Z: Department of Automatic Control and Systems Engineering, University of Sheffield, Sheffield, S1 3JD, UK; School of Information Science and Engineering, East China University of Science and Technology, Shanghai 200237, China
Wang B: School of Information Science and Engineering, East China University of Science and Technology, Shanghai 200237, China
Wang Y: School of Information Science and Engineering, East China University of Science and Technology, Shanghai 200237, China
Cao C: School of Information Science and Engineering, East China University of Science and Technology, Shanghai 200237, China [ORCID]
Peng X: School of Information Science and Engineering, East China University of Science and Technology, Shanghai 200237, China
Du W: School of Information Science and Engineering, East China University of Science and Technology, Shanghai 200237, China
Qian F: School of Information Science and Engineering, East China University of Science and Technology, Shanghai 200237, China
Wang B: School of Information Science and Engineering, East China University of Science and Technology, Shanghai 200237, China
Wang Y: School of Information Science and Engineering, East China University of Science and Technology, Shanghai 200237, China
Cao C: School of Information Science and Engineering, East China University of Science and Technology, Shanghai 200237, China [ORCID]
Peng X: School of Information Science and Engineering, East China University of Science and Technology, Shanghai 200237, China
Du W: School of Information Science and Engineering, East China University of Science and Technology, Shanghai 200237, China
Qian F: School of Information Science and Engineering, East China University of Science and Technology, Shanghai 200237, China
Journal Name
Processes
Volume
10
Issue
8
First Page
1633
Year
2022
Publication Date
2022-08-17
Published Version
ISSN
2227-9717
Version Comments
Original Submission
Other Meta
PII: pr10081633, Publication Type: Journal Article
Record Map
Published Article
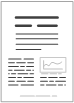
LAPSE:2023.1657
This Record
External Link
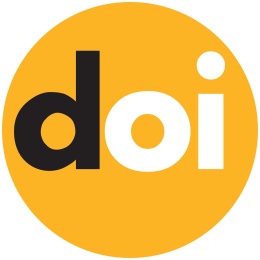
doi:10.3390/pr10081633
Publisher Version
Download
Meta
Record Statistics
Record Views
63
Version History
[v1] (Original Submission)
Feb 21, 2023
Verified by curator on
Feb 21, 2023
This Version Number
v1
Citations
Most Recent
This Version
URL Here
https://psecommunity.org/LAPSE:2023.1657
Original Submitter
Auto Uploader for LAPSE
Links to Related Works