LAPSE:2023.16077
Published Article
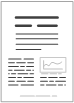
LAPSE:2023.16077
Accelerating Energy-Economic Simulation Models via Machine Learning-Based Emulation and Time Series Aggregation
March 2, 2023
Energy-economic simulation models with high levels of detail, high time resolutions, or large populations (e.g., distribution networks, households, electric vehicles, energy communities) are often limited due to their computational complexity. This paper introduces a novel methodology, combining cluster-based time series aggregation and sampling methods, to efficiently emulate simulation models using machine learning and significantly reduce both simulation and training time. Machine learning-based emulation models require sufficient and high-quality data to generalize the dataset. Since simulations are computationally complex, their maximum number is limited. Sampling methods come into play when selecting the best parameters for a limited number of simulations ex ante. This paper introduces and compares multiple sampling methods on three energy-economic datasets and shows their advantage over a simple random sampling for small sample-sizes. The results show that a k-means cluster sampling approach (based on unsupervised learning) and adaptive sampling (based on supervised learning) achieve the best results especially for small sample sizes. While a k-means cluster sampling is simple to implement, it is challenging to increase the sample sizes if the emulation model does not achieve sufficient accuracy. The iterative adaptive sampling is more complex during implementation, but can be re-applied until a certain accuracy threshold is met. Emulation is then applied on a case study, emulating an energy-economic simulation framework for peer-to-peer pricing models in Germany. The evaluated pricing models are the “supply and demand ratio” (SDR) and “mid-market rate pricing” (MMR). A time series aggregation can reduce time series data of municipalities by 99.4% with less than 5% error for 98.2% (load) and 95.5% (generation) of all municipalities and hence decrease the simulation time needed to create sufficient training data. This paper combines time series aggregation and emulation in a novel approach and shows significant acceleration by up to 88.9% of the model’s initial runtime for the simulation of the entire population of around 12,000 municipalities. The time for re-calculating the population (e.g., for different scenarios or sensitivity analysis) can be increased by a factor of 1100 while still retaining high accuracy. The analysis of the simulation time shows that time series aggregation and emulation, considered individually, only bring minor improvements in the runtime but can, however, be combined effectively. This can significantly speed up both the simulation itself and the training of the emulation model and allows for flexible use, depending on the capabilities of the models and the practitioners. The results of the peer-to-peer pricing for approximately 12,000 German municipalities show great potential for energy communities. The mechanisms offer good incentives for the addition of necessary flexibility.
Record ID
Keywords
Artificial Intelligence, distributed energy resources, electricity markets, emulation-model, energy communities, Machine Learning, meta-model, sampling, surrogate-model, TSA
Subject
Suggested Citation
Bogensperger AJ, Fabel Y, Ferstl J. Accelerating Energy-Economic Simulation Models via Machine Learning-Based Emulation and Time Series Aggregation. (2023). LAPSE:2023.16077
Author Affiliations
Bogensperger AJ: Forschungsstelle fuer Energiewirtschaft e.V. (FFE), Am Bluetenanger 71, 80995 Munich, Germany; TUM School of Engineering and Design, Technical University of Munich, Arcisstraße 21, 80333 Munich, Germany
Fabel Y: Forschungsstelle fuer Energiewirtschaft e.V. (FFE), Am Bluetenanger 71, 80995 Munich, Germany
Ferstl J: Forschungsstelle fuer Energiewirtschaft e.V. (FFE), Am Bluetenanger 71, 80995 Munich, Germany
Fabel Y: Forschungsstelle fuer Energiewirtschaft e.V. (FFE), Am Bluetenanger 71, 80995 Munich, Germany
Ferstl J: Forschungsstelle fuer Energiewirtschaft e.V. (FFE), Am Bluetenanger 71, 80995 Munich, Germany
Journal Name
Energies
Volume
15
Issue
3
First Page
1239
Year
2022
Publication Date
2022-02-08
Published Version
ISSN
1996-1073
Version Comments
Original Submission
Other Meta
PII: en15031239, Publication Type: Journal Article
Record Map
Published Article
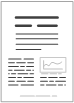
LAPSE:2023.16077
This Record
External Link
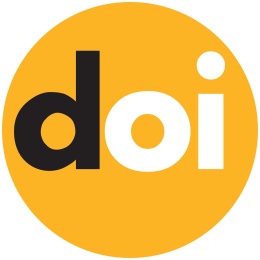
doi:10.3390/en15031239
Publisher Version
Download
Meta
Record Statistics
Record Views
87
Version History
[v1] (Original Submission)
Mar 2, 2023
Verified by curator on
Mar 2, 2023
This Version Number
v1
Citations
Most Recent
This Version
URL Here
https://psecommunity.org/LAPSE:2023.16077
Original Submitter
Auto Uploader for LAPSE
Links to Related Works