LAPSE:2023.14839
Published Article
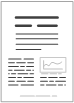
LAPSE:2023.14839
Research on Fuel Cell Fault Diagnosis Based on Genetic Algorithm Optimization of Support Vector Machine
March 1, 2023
The fuel cell engine mechanism model is used to research fault diagnosis based on a data-driven method to identify the failure of proton exchange membrane fuel cells in the process of operation, which leads to the degradation of system performance and other problems. In this paper, an extreme learning machine and a support vector machine are applied to classify the usual faults of fuel cells, including air compressor faults, air supply pipe and return pipe leaks, stack flooding faults and temperature controller faults. The accuracy of fault classification was 78.67% and 83.33% respectively. In order to improve the efficiency of fault classification, a genetic algorithm is used to optimize the parameters of the support vector machine. The simulation results show that the accuracy of fault classification was improved to 94% after optimization.
Record ID
Keywords
extreme learning machine, fault diagnosis, fuel cell, Genetic Algorithm, support vector machine
Subject
Suggested Citation
Huo W, Li W, Sun C, Ren Q, Gong G. Research on Fuel Cell Fault Diagnosis Based on Genetic Algorithm Optimization of Support Vector Machine. (2023). LAPSE:2023.14839
Author Affiliations
Huo W: School of Mechanical and Electrical Engineering, Beijing Information Science & Technology University, Beijing 100092, China; Collaborative Innovation Center of Electric Vehicles in Beijing, Beijing Information Science & Technology University, Beijing 1000
Li W: School of Mechanical and Electrical Engineering, Beijing Information Science & Technology University, Beijing 100092, China [ORCID]
Sun C: National Engineering Laboratory for Electric Vehicles, Beijing Institute of Technology, Beijing 100081, China
Ren Q: Guangzhou Automobile Group Co., Ltd., Automotive Engineering Research Institute, Guangzhou 510006, China
Gong G: School of Mechanical and Electrical Engineering, Beijing Information Science & Technology University, Beijing 100092, China
Li W: School of Mechanical and Electrical Engineering, Beijing Information Science & Technology University, Beijing 100092, China [ORCID]
Sun C: National Engineering Laboratory for Electric Vehicles, Beijing Institute of Technology, Beijing 100081, China
Ren Q: Guangzhou Automobile Group Co., Ltd., Automotive Engineering Research Institute, Guangzhou 510006, China
Gong G: School of Mechanical and Electrical Engineering, Beijing Information Science & Technology University, Beijing 100092, China
Journal Name
Energies
Volume
15
Issue
6
First Page
2294
Year
2022
Publication Date
2022-03-21
Published Version
ISSN
1996-1073
Version Comments
Original Submission
Other Meta
PII: en15062294, Publication Type: Journal Article
Record Map
Published Article
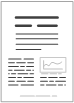
LAPSE:2023.14839
This Record
External Link
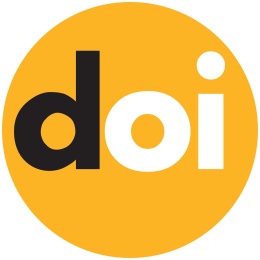
doi:10.3390/en15062294
Publisher Version
Download
Meta
Record Statistics
Record Views
79
Version History
[v1] (Original Submission)
Mar 1, 2023
Verified by curator on
Mar 1, 2023
This Version Number
v1
Citations
Most Recent
This Version
URL Here
https://psecommunity.org/LAPSE:2023.14839
Original Submitter
Auto Uploader for LAPSE
Links to Related Works