LAPSE:2023.14691
Published Article
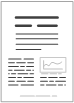
LAPSE:2023.14691
Forecasting of Electric Load Using a Hybrid LSTM-Neural Prophet Model
March 1, 2023
Load forecasting (LF) is an essential factor in power system management. LF helps the utility maximize the utilization of power-generating plants and schedule them both reliably and economically. In this paper, a novel and hybrid forecasting method is proposed, combining a long short-term memory network (LSTM) and neural prophet (NP) through an artificial neural network. The paper aims to predict electric load for different time horizons with improved accuracy as well as consistency. The proposed model uses historical load data, weather data, and statistical features obtained from the historical data. Multiple case studies have been conducted with two different real-time data sets on three different types of load forecasting. The hybrid model is later compared with a few established methods of load forecasting found in the literature with different performance metrics: mean average percentage error (MAPE), root mean square error (RMSE), sum of square error (SSE), and regression coefficient (R). Moreover, a guideline with various attributes is provided for different types of load forecasting considering the applications of the proposed model. The results and comparisons from our test cases showed that the proposed hybrid model improved the forecasting accuracy for three different types of load forecasting over other forecasting techniques.
Record ID
Keywords
Artificial Intelligence, artificial neural network, load forecasting, long short-term memory, neural prophet, time series forecasting
Suggested Citation
Shohan MJA, Faruque MO, Foo SY. Forecasting of Electric Load Using a Hybrid LSTM-Neural Prophet Model. (2023). LAPSE:2023.14691
Author Affiliations
Shohan MJA: Department of Electrical and Computer Engineering, FAMU-FSU College of Engineering, Florida State University, Tallahassee, FL 32310, USA; Center for Advanced Power Systems (CAPS), Florida State University, Tallahassee, FL 32310, USA [ORCID]
Faruque MO: Department of Electrical and Computer Engineering, FAMU-FSU College of Engineering, Florida State University, Tallahassee, FL 32310, USA; Center for Advanced Power Systems (CAPS), Florida State University, Tallahassee, FL 32310, USA [ORCID]
Foo SY: Department of Electrical and Computer Engineering, FAMU-FSU College of Engineering, Florida State University, Tallahassee, FL 32310, USA
Faruque MO: Department of Electrical and Computer Engineering, FAMU-FSU College of Engineering, Florida State University, Tallahassee, FL 32310, USA; Center for Advanced Power Systems (CAPS), Florida State University, Tallahassee, FL 32310, USA [ORCID]
Foo SY: Department of Electrical and Computer Engineering, FAMU-FSU College of Engineering, Florida State University, Tallahassee, FL 32310, USA
Journal Name
Energies
Volume
15
Issue
6
First Page
2158
Year
2022
Publication Date
2022-03-16
Published Version
ISSN
1996-1073
Version Comments
Original Submission
Other Meta
PII: en15062158, Publication Type: Journal Article
Record Map
Published Article
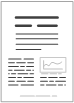
LAPSE:2023.14691
This Record
External Link
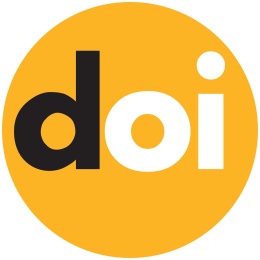
doi:10.3390/en15062158
Publisher Version
Download
Meta
Record Statistics
Record Views
81
Version History
[v1] (Original Submission)
Mar 1, 2023
Verified by curator on
Mar 1, 2023
This Version Number
v1
Citations
Most Recent
This Version
URL Here
https://psecommunity.org/LAPSE:2023.14691
Original Submitter
Auto Uploader for LAPSE
Links to Related Works