LAPSE:2023.1115
Published Article
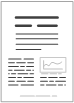
LAPSE:2023.1115
Introducing the Effective Features Using the Particle Swarm Optimization Algorithm to Increase Accuracy in Determining the Volume Percentages of Three-Phase Flows
February 21, 2023
What is presented in this research is an intelligent system for detecting the volume percentage of three-phase fluids passing through oil pipes. The structure of the detection system consists of an X-ray tube, a Pyrex galss pipe, and two sodium iodide detectors. A three-phase fluid of water, gas, and oil has been simulated inside the pipe in two flow regimes, annular and stratified. Different volume percentages from 10 to 80% are considered for each phase. After producing and emitting X-rays from the source and passing through the pipe containing a three-phase fluid, the intensity of photons is recorded by two detectors. The simulation is introduced by a Monte Carlo N-Particle (MCNP) code. After the implementation of all flow regimes in different volume percentages, the signals recorded by the detectors were recorded and labeled. Three frequency characteristics and five wavelet transform characteristics were extracted from the received signals of each detector, which were collected in a total of 16 characteristics from each test. The feature selection system based on the particle swarm optimization (PSO) algorithm was applied to determine the best combination of extracted features. The result was the introduction of seven features as the best features to determine volume percentages. The introduced characteristics were considered as the input of a Multilayer Perceptron (MLP) neural network, whose structure had seven input neurons (selected characteristics) and two output neurons (volume percentage of gas and water). The highest error obtained in determining volume percentages was equal to 0.13 as MSE, a low error compared with previous works. Using the PSO algorithm to select the most optimal features, the current research’s accuracy in determining volume percentages has significantly increased.
Record ID
Keywords
Artificial Intelligence, feature extraction, frequency domain, MLP neural network, PSO, volume fraction, wavelet
Suggested Citation
Chen TC, Alizadeh SM, Albahar MA, Thanoon M, Alammari A, Guerrero JWG, Nazemi E, Eftekhari-Zadeh E. Introducing the Effective Features Using the Particle Swarm Optimization Algorithm to Increase Accuracy in Determining the Volume Percentages of Three-Phase Flows. (2023). LAPSE:2023.1115
Author Affiliations
Chen TC: College of Management and Design, Ming Chi University of Technology, ROC, New Taipei City 243303, Taiwan [ORCID]
Alizadeh SM: Petroleum Engineering Department, Australian University, West Mishref 13015, Kuwait [ORCID]
Albahar MA: School of Computer Science, Umm Al-Qura University, Mecca 24382, Saudi Arabia
Thanoon M: School of Computer Science, Umm Al-Qura University, Mecca 24382, Saudi Arabia [ORCID]
Alammari A: Faculty of Education, Curriculums and Teaching Department, Umm Al-Qura University, Makkah 24382, Saudi Arabia
Guerrero JWG: Department of Energy, Universidad de la Costa, Barranquilla 080001, Colombia [ORCID]
Nazemi E: Imec-Vision Laboratory, University of Antwerp, 2610 Antwerp, Belgium [ORCID]
Eftekhari-Zadeh E: Institute of Optics and Quantum Electronics, Abbe Center of Photonics, Friedrich Schiller University Jena, 07743 Jena, Germany [ORCID]
Alizadeh SM: Petroleum Engineering Department, Australian University, West Mishref 13015, Kuwait [ORCID]
Albahar MA: School of Computer Science, Umm Al-Qura University, Mecca 24382, Saudi Arabia
Thanoon M: School of Computer Science, Umm Al-Qura University, Mecca 24382, Saudi Arabia [ORCID]
Alammari A: Faculty of Education, Curriculums and Teaching Department, Umm Al-Qura University, Makkah 24382, Saudi Arabia
Guerrero JWG: Department of Energy, Universidad de la Costa, Barranquilla 080001, Colombia [ORCID]
Nazemi E: Imec-Vision Laboratory, University of Antwerp, 2610 Antwerp, Belgium [ORCID]
Eftekhari-Zadeh E: Institute of Optics and Quantum Electronics, Abbe Center of Photonics, Friedrich Schiller University Jena, 07743 Jena, Germany [ORCID]
Journal Name
Processes
Volume
11
Issue
1
First Page
236
Year
2023
Publication Date
2023-01-11
Published Version
ISSN
2227-9717
Version Comments
Original Submission
Other Meta
PII: pr11010236, Publication Type: Journal Article
Record Map
Published Article
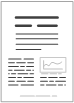
LAPSE:2023.1115
This Record
External Link
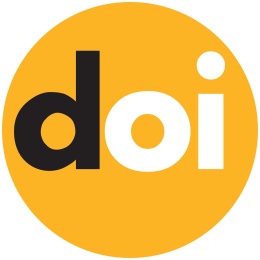
doi:10.3390/pr11010236
Publisher Version
Download
Meta
Record Statistics
Record Views
85
Version History
[v1] (Original Submission)
Feb 21, 2023
Verified by curator on
Feb 21, 2023
This Version Number
v1
Citations
Most Recent
This Version
URL Here
https://psecommunity.org/LAPSE:2023.1115
Original Submitter
Auto Uploader for LAPSE
Links to Related Works