LAPSE:2023.10973
Published Article
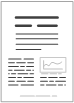
LAPSE:2023.10973
Deep-Learning Based Fault Events Analysis in Power Systems
February 27, 2023
The identification of fault types and their locations is crucial for power system protection/operation when a fault occurs in the lines. In general, this involves a human-in-the-loop analysis to capture the transient voltage and current signals using a common format for transient data exchange for power systems (COMTRADE) file. Then, protection engineers can identify the fault types and the line locations after the incident. This paper proposes intelligent and novel methods of faulty line and location detection based on convolutional neural networks in the power system. The three-phase fault information contained in the COMTRADE file is converted to an image file and extracted adaptively by the proposed CNN, which is trained by a large number of images under various kinds of fault conditions and factors. A 500 kV power system is simulated to generate different types of electromagnetic fault transients. The test results show that the proposed CNN-based analyzer can classify the fault types and locations under various conditions and reduce the fault analysis efforts.
Record ID
Keywords
convolutional neural networks, fault line location identification, power systems fault classification
Subject
Suggested Citation
Hong J, Kim YH, Nhung-Nguyen H, Kwon J, Lee H. Deep-Learning Based Fault Events Analysis in Power Systems. (2023). LAPSE:2023.10973
Author Affiliations
Hong J: Department of Electrical and Computer Engineering, University of Michigan-Dearborn, Dearborn, MI 48128, USA [ORCID]
Kim YH: Department of Data Science, Korea National University of Transportation, Uiwang-si 16106, Korea [ORCID]
Nhung-Nguyen H: Department of Electronic Engineering, Myongji University, Yongin-si 17508, Korea; Department of Information Technology, Viet Tri University of Industry, Viet Tri 29000, Vietnam
Kwon J: Department of Electrical and Computer Engineering, University of Michigan-Dearborn, Dearborn, MI 48128, USA [ORCID]
Lee H: Hitachi Energy, Raleigh, NC 27606, USA
Kim YH: Department of Data Science, Korea National University of Transportation, Uiwang-si 16106, Korea [ORCID]
Nhung-Nguyen H: Department of Electronic Engineering, Myongji University, Yongin-si 17508, Korea; Department of Information Technology, Viet Tri University of Industry, Viet Tri 29000, Vietnam
Kwon J: Department of Electrical and Computer Engineering, University of Michigan-Dearborn, Dearborn, MI 48128, USA [ORCID]
Lee H: Hitachi Energy, Raleigh, NC 27606, USA
Journal Name
Energies
Volume
15
Issue
15
First Page
5539
Year
2022
Publication Date
2022-07-30
Published Version
ISSN
1996-1073
Version Comments
Original Submission
Other Meta
PII: en15155539, Publication Type: Journal Article
Record Map
Published Article
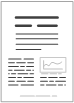
LAPSE:2023.10973
This Record
External Link
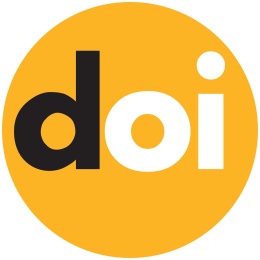
doi:10.3390/en15155539
Publisher Version
Download
Meta
Record Statistics
Record Views
103
Version History
[v1] (Original Submission)
Feb 27, 2023
Verified by curator on
Feb 27, 2023
This Version Number
v1
Citations
Most Recent
This Version
URL Here
https://psecommunity.org/LAPSE:2023.10973
Original Submitter
Auto Uploader for LAPSE
Links to Related Works