LAPSE:2023.10329
Published Article
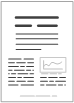
LAPSE:2023.10329
FDD in Building Systems Based on Generalized Machine Learning Approaches
February 27, 2023
Automated fault detection and diagnostics in building systems using machine learning (ML) can be applied to commercial buildings and can result in increased efficiency and savings. Using ML for FDD brings the benefit of advancing the analytics of a building. An automated process was developed to provide ML-based building analytics to building engineers and operators with minimal training. The process can be applied to buildings with a variety of configurations, which saves time and manual effort in a fault analysis. Classification analysis is used for fault detection and diagnostics. An ML analysis is defined which introduces advanced diagnostics with metrics to quantify a fault’s impact in the system and rank detected faults in order of impact severity. Explanations of the methodology used for the ML analysis include a description of the algorithms used. The analysis was applied to a building on the Texas A&M University campus where the results are shown to illustrate the performance of the process using measured data from a building.
Record ID
Keywords
building systems, Fault Detection, fault diagnosis, HVAC, Machine Learning
Subject
Suggested Citation
Nelson W, Culp C. FDD in Building Systems Based on Generalized Machine Learning Approaches. (2023). LAPSE:2023.10329
Author Affiliations
Nelson W: Department of Mechanical Engineering, Energy Systems Laboratory, Texas AM University, College Station, TX 77843, USA
Culp C: Department of Architecture, Energy Systems Laboratory, Texas AM University, College Station, TX 77843, USA [ORCID]
Culp C: Department of Architecture, Energy Systems Laboratory, Texas AM University, College Station, TX 77843, USA [ORCID]
Journal Name
Energies
Volume
16
Issue
4
First Page
1637
Year
2023
Publication Date
2023-02-07
Published Version
ISSN
1996-1073
Version Comments
Original Submission
Other Meta
PII: en16041637, Publication Type: Journal Article
Record Map
Published Article
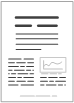
LAPSE:2023.10329
This Record
External Link
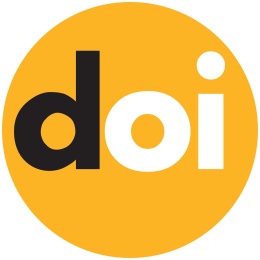
doi:10.3390/en16041637
Publisher Version
Download
Meta
Record Statistics
Record Views
74
Version History
[v1] (Original Submission)
Feb 27, 2023
Verified by curator on
Feb 27, 2023
This Version Number
v1
Citations
Most Recent
This Version
URL Here
https://psecommunity.org/LAPSE:2023.10329
Original Submitter
Auto Uploader for LAPSE
Links to Related Works