LAPSE:2023.10130
Published Article
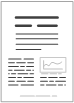
LAPSE:2023.10130
Fault Detection and Identification of Furnace Negative Pressure System with CVA and GA-XGBoost
February 27, 2023
The boiler is an essential energy conversion facility in a thermal power plant. One small malfunction or abnormal event will bring huge economic loss and casualties. Accurate and timely detection of abnormal events in boilers is crucial for the safe and economical operation of complex thermal power plants. Data-driven fault diagnosis methods based on statistical process monitoring technology have prevailed in thermal power plants, whereas the false alarm rates of those methods are relatively high. To work around this, this paper proposes a novel fault detection and identification method for furnace negative pressure system based on canonical variable analysis (CVA) and eXtreme Gradient Boosting improved by genetic algorithms (GA-XGBoost). First, CVA is used to reduce the data redundancy and construct the canonical residuals to measure the prediction ability of the state variables. Then, the fault detection model based on GA-XGBoost is schemed using the constructed canonical residual variables. Specially, GA is introduced to determine the optimal hyperparameters of XGBoost and speed up the convergence. Next, this paper presents a novel fault identification method based on the reconstructed contribution statistics, considering the contribution of state space, residual space and canonical residual space. Besides, the proposed statistics renders different weights to the state vectors, the residual vectors and the canonical residual vectors to improve the sensitivity of faulty variables. Finally, the real industrial data from a boiler furnace negative pressure system of a certain thermal power plant is used to demonstrate the ability of the proposed method. The result demonstrates that this method is accurate and efficient to detect and identify the faults of a true boiler.
Record ID
Keywords
canonical variable residual analysis, Fault Detection, furnace negative pressure, reconstructed variable contribution, XGBoost
Subject
Suggested Citation
Ling D, Li C, Wang Y, Zhang P. Fault Detection and Identification of Furnace Negative Pressure System with CVA and GA-XGBoost. (2023). LAPSE:2023.10130
Author Affiliations
Ling D: School of Electrical and Information Engineering, Zhengzhou University of Light Industry, Zhengzhou 450000, China [ORCID]
Li C: School of Electrical and Information Engineering, Zhengzhou University of Light Industry, Zhengzhou 450000, China
Wang Y: School of Electrical and Information Engineering, Zhengzhou University of Light Industry, Zhengzhou 450000, China [ORCID]
Zhang P: School of Electrical and Information Engineering, Zhengzhou University of Light Industry, Zhengzhou 450000, China
Li C: School of Electrical and Information Engineering, Zhengzhou University of Light Industry, Zhengzhou 450000, China
Wang Y: School of Electrical and Information Engineering, Zhengzhou University of Light Industry, Zhengzhou 450000, China [ORCID]
Zhang P: School of Electrical and Information Engineering, Zhengzhou University of Light Industry, Zhengzhou 450000, China
Journal Name
Energies
Volume
15
Issue
17
First Page
6355
Year
2022
Publication Date
2022-08-31
Published Version
ISSN
1996-1073
Version Comments
Original Submission
Other Meta
PII: en15176355, Publication Type: Journal Article
Record Map
Published Article
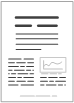
LAPSE:2023.10130
This Record
External Link
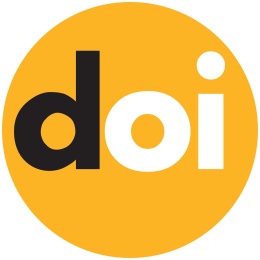
doi:10.3390/en15176355
Publisher Version
Download
Meta
Record Statistics
Record Views
76
Version History
[v1] (Original Submission)
Feb 27, 2023
Verified by curator on
Feb 27, 2023
This Version Number
v1
Citations
Most Recent
This Version
URL Here
https://psecommunity.org/LAPSE:2023.10130
Original Submitter
Auto Uploader for LAPSE
Links to Related Works