LAPSE:2023.0123
Published Article
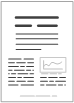
LAPSE:2023.0123
An Integrated Method of Bayesian Optimization and D-Optimal Design for Chemical Experiment Optimization
February 17, 2023
The smart chemical laboratory has recently emerged as a promising trend for future chemical research, where experiment optimization is of vital importance. The traditional Bayesian optimization (BO) algorithm focuses on exploring the dependent variable space while overlooking the independent variable space. Consequently, the BO algorithm suffers from becoming stuck at local optima, which severely deteriorates the optimization performance, especially with bad-quality initial points. Herein, we propose a novel stochastic framework of Bayesian optimization with D-optimal design (BODO) by integrating BO with D-optimal design. BODO can balance the exploitation in the dependent variable space and the exploration in the independent variable space. We highlight the excellent performance of BODO even with poor initial points on the benchmark alpine2 function. Meanwhile, BODO demonstrates a better average objective function value than BO on the benchmark Summit SnAr chemical process, showing its advantage in chemical experiment optimization and potential application in future chemical experiments.
Record ID
Keywords
Bayesian optimization, chemical experiment optimization, D-optimal design, experiment space exploration
Subject
Suggested Citation
Huang X, Zhang S, Li H, Qiu T. An Integrated Method of Bayesian Optimization and D-Optimal Design for Chemical Experiment Optimization. (2023). LAPSE:2023.0123
Author Affiliations
Huang X: Department of Chemical Engineering, Tsinghua University, Beijing 100084, China; Beijing Key Laboratory of Industrial Big Data Systems and Applications, Tsinghua University, Beijing 100084, China [ORCID]
Zhang S: Department of Chemical Engineering, Tsinghua University, Beijing 100084, China; Beijing Key Laboratory of Industrial Big Data Systems and Applications, Tsinghua University, Beijing 100084, China [ORCID]
Li H: Department of Chemical Engineering, Tsinghua University, Beijing 100084, China; Beijing Key Laboratory of Industrial Big Data Systems and Applications, Tsinghua University, Beijing 100084, China [ORCID]
Qiu T: Department of Chemical Engineering, Tsinghua University, Beijing 100084, China; Beijing Key Laboratory of Industrial Big Data Systems and Applications, Tsinghua University, Beijing 100084, China [ORCID]
Zhang S: Department of Chemical Engineering, Tsinghua University, Beijing 100084, China; Beijing Key Laboratory of Industrial Big Data Systems and Applications, Tsinghua University, Beijing 100084, China [ORCID]
Li H: Department of Chemical Engineering, Tsinghua University, Beijing 100084, China; Beijing Key Laboratory of Industrial Big Data Systems and Applications, Tsinghua University, Beijing 100084, China [ORCID]
Qiu T: Department of Chemical Engineering, Tsinghua University, Beijing 100084, China; Beijing Key Laboratory of Industrial Big Data Systems and Applications, Tsinghua University, Beijing 100084, China [ORCID]
Journal Name
Processes
Volume
11
Issue
1
First Page
87
Year
2022
Publication Date
2022-12-28
Published Version
ISSN
2227-9717
Version Comments
Original Submission
Other Meta
PII: pr11010087, Publication Type: Journal Article
Record Map
Published Article
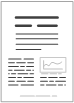
LAPSE:2023.0123
This Record
External Link
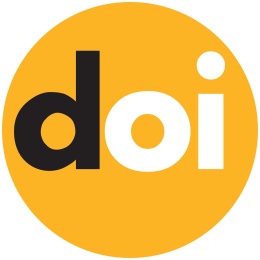
doi:10.3390/pr11010087
Publisher Version
Download
Meta
Record Statistics
Record Views
66
Version History
[v1] (Original Submission)
Feb 17, 2023
Verified by curator on
Feb 17, 2023
This Version Number
v1
Citations
Most Recent
This Version
URL Here
https://psecommunity.org/LAPSE:2023.0123
Original Submitter
Auto Uploader for LAPSE
Links to Related Works