LAPSE:2020.1054
Published Article
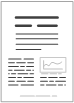
LAPSE:2020.1054
A Novel Bearing Fault Diagnosis Method Based on GL-mRMR-SVM
October 26, 2020
A convolutional neural network (CNN) has been used to successfully realize end-to-end bearing fault diagnosis due to its powerful feature extraction ability. However, the CNN is prone to focus on local information, ignoring the relationship between the whole and the part of the signal due to its unique structure. In addition, it extracts some fault features with poor robustness under noisy environment. A novel diagnosis model based on feature fusion and feature selection, GL-mRMR-SVM, is proposed to address this problem in this paper. First, the model combines the global features in the time-domain and frequency-domain of the raw data with the local features extracted by CNN to make full use of the signal information and overcome the weakness of traditional CNNs neglecting the overall signal. Then, the max-relevance min-redundancy (mRMR) algorithm is used to automatically extract the discriminative features from the fused features without any prior knowledge. Finally, the extracted discriminative features are input into the SVM for training and output the fault recognition results. The proposed GL-mRMR-SVM model was evaluated through experiments on bearing data of Case Western Reserve University (CWRU) and CUT-2 platform. The experimental results show that the proposed method is more effective than other intelligent diagnosis methods.
Record ID
Keywords
bearing fault diagnosis, convolutional neural network (CNN), global feature, local feature, max-relevance min-redundancy (mRMR)
Subject
Suggested Citation
Tang X, He Q, Gu X, Li C, Zhang H, Lu J. A Novel Bearing Fault Diagnosis Method Based on GL-mRMR-SVM. (2020). LAPSE:2020.1054
Author Affiliations
Tang X: Key Laboratory of Advanced Manufacturing Technology, Ministry of Education, Guizhou University, Guiyang 550025, China; School of Mechanical Engineering, Guizhou University, Guiyang 550025, China; Guizhou Provincial Key Laboratory of Public Big Data Guizho
He Q: Key Laboratory of Advanced Manufacturing Technology, Ministry of Education, Guizhou University, Guiyang 550025, China [ORCID]
Gu X: Key Laboratory of Advanced Manufacturing Technology, Ministry of Education, Guizhou University, Guiyang 550025, China
Li C: Key Laboratory of Advanced Manufacturing Technology, Ministry of Education, Guizhou University, Guiyang 550025, China [ORCID]
Zhang H: Key Laboratory of Advanced Manufacturing Technology, Ministry of Education, Guizhou University, Guiyang 550025, China [ORCID]
Lu J: Key Laboratory of Advanced Manufacturing Technology, Ministry of Education, Guizhou University, Guiyang 550025, China; School of Mechanical Engineering, Guizhou University, Guiyang 550025, China; Guizhou Provincial Key Laboratory of Public Big Data Guizho
He Q: Key Laboratory of Advanced Manufacturing Technology, Ministry of Education, Guizhou University, Guiyang 550025, China [ORCID]
Gu X: Key Laboratory of Advanced Manufacturing Technology, Ministry of Education, Guizhou University, Guiyang 550025, China
Li C: Key Laboratory of Advanced Manufacturing Technology, Ministry of Education, Guizhou University, Guiyang 550025, China [ORCID]
Zhang H: Key Laboratory of Advanced Manufacturing Technology, Ministry of Education, Guizhou University, Guiyang 550025, China [ORCID]
Lu J: Key Laboratory of Advanced Manufacturing Technology, Ministry of Education, Guizhou University, Guiyang 550025, China; School of Mechanical Engineering, Guizhou University, Guiyang 550025, China; Guizhou Provincial Key Laboratory of Public Big Data Guizho
Journal Name
Processes
Volume
8
Issue
7
Article Number
E784
Year
2020
Publication Date
2020-07-05
Published Version
ISSN
2227-9717
Version Comments
Original Submission
Other Meta
PII: pr8070784, Publication Type: Journal Article
Record Map
Published Article
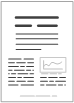
LAPSE:2020.1054
This Record
External Link
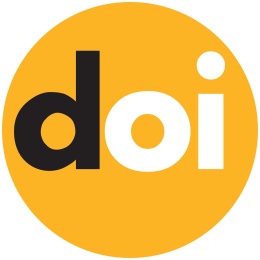
doi:10.3390/pr8070784
Publisher Version
Download
Meta
Record Statistics
Record Views
352
Version History
[v1] (Original Submission)
Oct 26, 2020
Verified by curator on
Oct 26, 2020
This Version Number
v1
Citations
Most Recent
This Version
URL Here
https://psecommunity.org/LAPSE:2020.1054
Original Submitter
Calvin Tsay
Links to Related Works