LAPSE:2020.0878
Published Article
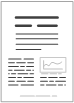
LAPSE:2020.0878
Research on State Recognition and Failure Prediction of Axial Piston Pump Based on Performance Degradation Data
July 17, 2020
Degradation state recognition and failure prediction are the key steps of prognostic and health management (PHM), which directly affect the reliability of the equipment and the selection of preventive maintenance strategy. Given the problem that the distinction between feature vectors is not obvious and the accuracy of fault prediction is low, a method based on multi-class Gaussian process classification and Gaussian process regression (GPR) is studied by the vibration signal and flow signal in six degraded states of the axial piston pump. For degradation state recognition, the variational mode decomposition (VMD) was used to decompose the vibration signal, and obtaining intrinsic mode function (IMF) components with rich information. Subsequently, multi-scale permutation entropy (MPE) was employed to select feature vectors of IMF components in different states. In order to reduce feature dimensions and improve recognition performance, ReliefF was used to select feature vectors with high weight, then a method based on multi-class Gaussian process classification was established by using these feature vectors to realize the research on the degradation state recognition. The test results demonstrate that the method can effectively identify the degradation state. Its recognition rate reaches 98.9%. Besides, for failure prediction, through the analysis of the wear process and wear mechanism of the valve plate, the curve fitting between the flow and the wear amount was performed by GPR to realize the failure prediction of the axial piston pump. Depending on the evaluation index, the GPR obtained a better failure prediction effect. The results will assist in the realization of predictive maintenance, and which also has significant practical value in project items.
Record ID
Keywords
axial piston pump, degraded state recognition, failure prediction, Gaussian process regression, multi-class Gaussian process classification, multi-scale permutation entropy
Subject
Suggested Citation
Guo R, Zhao Z, Huo S, Jin Z, Zhao J, Gao D. Research on State Recognition and Failure Prediction of Axial Piston Pump Based on Performance Degradation Data. (2020). LAPSE:2020.0878
Author Affiliations
Guo R: Hebei Provincial Key Laboratory of Heavy Machinery Fluid Power Transmission and Control, Yanshan University, Qinhuangdao 066004, China; The State Key Laboratory of Fluid Power and Mechatronic Systems, Zhejiang University, Hangzhou 310027, China [ORCID]
Zhao Z: Hebei Provincial Key Laboratory of Heavy Machinery Fluid Power Transmission and Control, Yanshan University, Qinhuangdao 066004, China; Key Laboratory of Advanced Forging and Stamping Technology and Science, Yanshan University, Qinhuangdao 066004, China
Huo S: Hebei Provincial Key Laboratory of Heavy Machinery Fluid Power Transmission and Control, Yanshan University, Qinhuangdao 066004, China
Jin Z: Key Laboratory of Advanced Forging and Stamping Technology and Science, Yanshan University, Qinhuangdao 066004, China
Zhao J: The State Key Laboratory of Fluid Power and Mechatronic Systems, Zhejiang University, Hangzhou 310027, China; Hebei Key Laboratory of Special Delivery Equipment, Yanshan University, Qinhuangdao 066004, China
Gao D: Hebei Provincial Key Laboratory of Heavy Machinery Fluid Power Transmission and Control, Yanshan University, Qinhuangdao 066004, China; Hebei Key Laboratory of Special Delivery Equipment, Yanshan University, Qinhuangdao 066004, China
Zhao Z: Hebei Provincial Key Laboratory of Heavy Machinery Fluid Power Transmission and Control, Yanshan University, Qinhuangdao 066004, China; Key Laboratory of Advanced Forging and Stamping Technology and Science, Yanshan University, Qinhuangdao 066004, China
Huo S: Hebei Provincial Key Laboratory of Heavy Machinery Fluid Power Transmission and Control, Yanshan University, Qinhuangdao 066004, China
Jin Z: Key Laboratory of Advanced Forging and Stamping Technology and Science, Yanshan University, Qinhuangdao 066004, China
Zhao J: The State Key Laboratory of Fluid Power and Mechatronic Systems, Zhejiang University, Hangzhou 310027, China; Hebei Key Laboratory of Special Delivery Equipment, Yanshan University, Qinhuangdao 066004, China
Gao D: Hebei Provincial Key Laboratory of Heavy Machinery Fluid Power Transmission and Control, Yanshan University, Qinhuangdao 066004, China; Hebei Key Laboratory of Special Delivery Equipment, Yanshan University, Qinhuangdao 066004, China
Journal Name
Processes
Volume
8
Issue
5
Article Number
E609
Year
2020
Publication Date
2020-05-20
Published Version
ISSN
2227-9717
Version Comments
Original Submission
Other Meta
PII: pr8050609, Publication Type: Journal Article
Record Map
Published Article
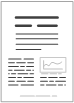
LAPSE:2020.0878
This Record
External Link
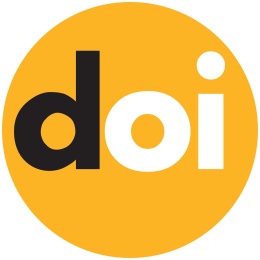
doi:10.3390/pr8050609
Publisher Version
Download
Meta
Record Statistics
Record Views
381
Version History
[v1] (Original Submission)
Jul 17, 2020
Verified by curator on
Jul 17, 2020
This Version Number
v1
Citations
Most Recent
This Version
URL Here
https://psecommunity.org/LAPSE:2020.0878
Original Submitter
Calvin Tsay
Links to Related Works