LAPSE:2018.0800
Published Article
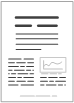
LAPSE:2018.0800
A Comparison of Energy Consumption Prediction Models Based on Neural Networks of a Bioclimatic Building
October 23, 2018
Energy consumption has been increasing steadily due to globalization and industrialization. Studies have shown that buildings are responsible for the biggest proportion of energy consumption; for example in European Union countries, energy consumption in buildings represents around 40% of the total energy consumption. In order to control energy consumption in buildings, different policies have been proposed, from utilizing bioclimatic architectures to the use of predictive models within control approaches. There are mainly three groups of predictive models including engineering, statistical and artificial intelligence models. Nowadays, artificial intelligence models such as neural networks and support vector machines have also been proposed because of their high potential capabilities of performing accurate nonlinear mappings between inputs and outputs in real environments which are not free of noise. The main objective of this paper is to compare a neural network model which was designed utilizing statistical and analytical methods, with a group of neural network models designed benefiting from a multi objective genetic algorithm. Moreover, the neural network models were compared to a naïve autoregressive baseline model. The models are intended to predict electric power demand at the Solar Energy Research Center (Centro de Investigación en Energía SOLar or CIESOL in Spanish) bioclimatic building located at the University of Almeria, Spain. Experimental results show that the models obtained from the multi objective genetic algorithm (MOGA) perform comparably to the model obtained through a statistical and analytical approach, but they use only 0.8% of data samples and have lower model complexity.
Record ID
Keywords
data selection, electric power demand, multi objective genetic algorithm (MOGA), neural networks, predictive model
Subject
Suggested Citation
Khosravani HR, Castilla MDM, Berenguel M, Ruano AE, Ferreira PM. A Comparison of Energy Consumption Prediction Models Based on Neural Networks of a Bioclimatic Building. (2018). LAPSE:2018.0800
Author Affiliations
Khosravani HR: Faculty of Science and Technology, University of Algarve, Campus Gambelas, Faro, Portugal; Institute of Mechanical Engineering (IDMEC), Instituto Superior Técnico, Universidade de Lisboa, Lisboa, Portugal
Castilla MDM: Department of Computer Science, Automatic Control, Robotics and Mechatronics Research Group, University of Almería, Agrifood Campus of International Excellence (ceiA3), CIESOL, Joint Center University of Almería-CIEMAT, Almería, Spain
Berenguel M: Department of Computer Science, Automatic Control, Robotics and Mechatronics Research Group, University of Almería, Agrifood Campus of International Excellence (ceiA3), CIESOL, Joint Center University of Almería-CIEMAT, Almería, Spain [ORCID]
Ruano AE: Faculty of Science and Technology, University of Algarve, Campus Gambelas, Faro, Portugal; Institute of Mechanical Engineering (IDMEC), Instituto Superior Técnico, Universidade de Lisboa, Lisboa, Portugal [ORCID]
Ferreira PM: LaSIGE, Faculdade de Ciências, Universidade de Lisboa, Portugal [ORCID]
[Login] to see author email addresses.
Castilla MDM: Department of Computer Science, Automatic Control, Robotics and Mechatronics Research Group, University of Almería, Agrifood Campus of International Excellence (ceiA3), CIESOL, Joint Center University of Almería-CIEMAT, Almería, Spain
Berenguel M: Department of Computer Science, Automatic Control, Robotics and Mechatronics Research Group, University of Almería, Agrifood Campus of International Excellence (ceiA3), CIESOL, Joint Center University of Almería-CIEMAT, Almería, Spain [ORCID]
Ruano AE: Faculty of Science and Technology, University of Algarve, Campus Gambelas, Faro, Portugal; Institute of Mechanical Engineering (IDMEC), Instituto Superior Técnico, Universidade de Lisboa, Lisboa, Portugal [ORCID]
Ferreira PM: LaSIGE, Faculdade de Ciências, Universidade de Lisboa, Portugal [ORCID]
[Login] to see author email addresses.
Journal Name
Energies
Volume
9
Issue
1
Article Number
E57
Year
2016
Publication Date
2016-01-20
Published Version
ISSN
1996-1073
Version Comments
Original Submission
Other Meta
PII: en9010057, Publication Type: Journal Article
Record Map
Published Article
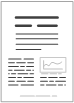
LAPSE:2018.0800
This Record
External Link
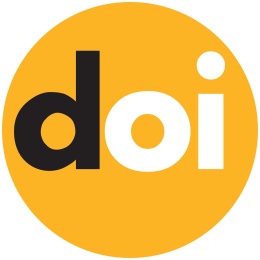
doi:10.3390/en9010057
Publisher Version
Download
Meta
Record Statistics
Record Views
770
Version History
[v1] (Original Submission)
Oct 23, 2018
Verified by curator on
Oct 23, 2018
This Version Number
v1
Citations
Most Recent
This Version
URL Here
https://psecommunity.org/LAPSE:2018.0800
Original Submitter
Calvin Tsay
Links to Related Works