LAPSE:2018.0762
Published Article
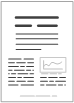
LAPSE:2018.0762
A Robust Weighted Combination Forecasting Method Based on Forecast Model Filtering and Adaptive Variable Weight Determination
October 23, 2018
Medium-and-long-term load forecasting plays an important role in energy policy implementation and electric department investment decision. Aiming to improve the robustness and accuracy of annual electric load forecasting, a robust weighted combination load forecasting method based on forecast model filtering and adaptive variable weight determination is proposed. Similar years of selection is carried out based on the similarity between the history year and the forecast year. The forecast models are filtered to select the better ones according to their comprehensive validity degrees. To determine the adaptive variable weight of the selected forecast models, the disturbance variable is introduced into Immune Algorithm-Particle Swarm Optimization (IA-PSO) and the adaptive adjustable strategy of particle search speed is established. Based on the forecast model weight determined by improved IA-PSO, the weighted combination forecast of annual electric load is obtained. The given case study illustrates the correctness and feasibility of the proposed method.
Record ID
Keywords
combination forecast, immune algorithm, load forecasting, Markov chain, normal cloud model, Particle Swarm Optimization, robustness
Subject
Suggested Citation
Li L, Mu C, Ding S, Wang Z, Mo R, Song Y. A Robust Weighted Combination Forecasting Method Based on Forecast Model Filtering and Adaptive Variable Weight Determination. (2018). LAPSE:2018.0762
Author Affiliations
Li L: College of Mechatronic Engineering, Beifang University of Nationalities, Yinchuan 750021, China
Mu C: State Key Laboratory of Robotics and System, Harbin Institute of Technology (HIT), Harbin 150001, China
Ding S: College of Mechatronic Engineering, Beifang University of Nationalities, Yinchuan 750021, China
Wang Z: State Grid Ningxia Electric Power Design Co. Ltd., Yinchuan 750001, China
Mo R: School of Management, Qingdao Technological University, Qingdao 266520, China; College of Electrical &Information Engineering, Hunan University, Changsha 410082, China
Song Y: School of Management, Qingdao Technological University, Qingdao 266520, China; College of Electrical Engineering and Information, Sichuan University, Chengdu 610065, China
[Login] to see author email addresses.
Mu C: State Key Laboratory of Robotics and System, Harbin Institute of Technology (HIT), Harbin 150001, China
Ding S: College of Mechatronic Engineering, Beifang University of Nationalities, Yinchuan 750021, China
Wang Z: State Grid Ningxia Electric Power Design Co. Ltd., Yinchuan 750001, China
Mo R: School of Management, Qingdao Technological University, Qingdao 266520, China; College of Electrical &Information Engineering, Hunan University, Changsha 410082, China
Song Y: School of Management, Qingdao Technological University, Qingdao 266520, China; College of Electrical Engineering and Information, Sichuan University, Chengdu 610065, China
[Login] to see author email addresses.
Journal Name
Energies
Volume
9
Issue
1
Article Number
E20
Year
2015
Publication Date
2015-12-31
Published Version
ISSN
1996-1073
Version Comments
Original Submission
Other Meta
PII: en9010020, Publication Type: Journal Article
Record Map
Published Article
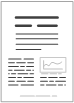
LAPSE:2018.0762
This Record
External Link
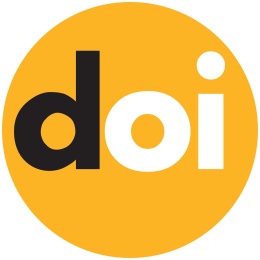
doi:10.3390/en9010020
Publisher Version
Download
Meta
Record Statistics
Record Views
609
Version History
[v1] (Original Submission)
Oct 23, 2018
Verified by curator on
Oct 23, 2018
This Version Number
v1
Citations
Most Recent
This Version
URL Here
https://psecommunity.org/LAPSE:2018.0762
Original Submitter
Calvin Tsay
Links to Related Works