LAPSE:2021.0798
Preprint
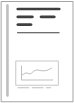
LAPSE:2021.0798
Optimization under uncertainty of a hybrid waste tire and natural gas feedstock flexible polygeneration system using a decomposition algorithm
April 5, 2022. Originally submitted on November 1, 2021
Market uncertainties motivate the development of flexible polygeneration systems that are able to adjust operating conditions to favor production of the most profitable product portfolio. However, this operational flexibility comes at the cost of higher capital expenditure. A scenario-based two-stage stochastic nonconvex Mixed-Integer Nonlinear Programming (MINLP) approach lends itself naturally to optimizing these trade-offs. This work studies the optimal design and operation under uncertainty of a hybrid feedstock flexible polygeneration system producing electricity, methanol, dimethyl ether, olefins or liquefied (synthetic) natural gas. The recently developed GOSSIP software framework is used for modeling the optimization problem as well as its efficient solution using the Nonconvex Generalized Benders Decomposition (NGBD) algorithm. Two different cases are studied: The first uses estimates of the means and variances of the uncertain parameters from historical data, whereas the second assesses the impact of increased uncertain parameter volatility. The value of implementing flexible designs characterized by the value of the stochastic solution (VSS) is in the range of 260 - 405 M$ for a scale of approximately 893 MW of thermal input. Increased price volatility around the same mean results in higher expected net present value and VSS as operational flexibility allows for asymmetric exploitation of price peaks.
Record ID
Keywords
Decomposition Algorithm, Optimization under uncertainty, Polygeneration system, Stochastic Programming, Waste Tire, Waste-to-Energy
Subject
Suggested Citation
Subramanian A, Kannan R, Holtorf F, Adams TA II, Gundersen T, Barton PI. Optimization under uncertainty of a hybrid waste tire and natural gas feedstock flexible polygeneration system using a decomposition algorithm. (2022). LAPSE:2021.0798
Author Affiliations
Subramanian A: Norwegian University of Science and Technology
Kannan R: Los Alamos National Laboratory
Holtorf F: Massachusetts Institute of Technology
Adams TA II: McMaster University
Gundersen T: Norwegian University of Science and Technology
Barton PI: Massachusetts Institute of Technology
[Login] to see author email addresses.
Kannan R: Los Alamos National Laboratory
Holtorf F: Massachusetts Institute of Technology
Adams TA II: McMaster University
Gundersen T: Norwegian University of Science and Technology
Barton PI: Massachusetts Institute of Technology
[Login] to see author email addresses.
Year
0
Version Comments
Included description of NGBD and Supplementary Material for completeness
Download
Meta
Record Statistics
Record Views
1057
Version History
[v2] (Included description of NGBD and Supplem...)
Apr 5, 2022
[v1] (Original Submission)
Nov 1, 2021
Verified by curator on
Nov 18, 2021
This Version Number
v2
Citations
Most Recent
This Version
URL Here
https://psecommunity.org/LAPSE:2021.0798
Original Submitter
a0088599