LAPSE:2019.1199
Published Article
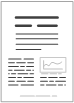
LAPSE:2019.1199
Handling Constraints and Raw Material Variability in Rotomolding through Data-Driven Model Predictive Control
November 24, 2019
This work addresses the problems of uniquely specifying and robustly achieving user-specified product quality in a complex industrial batch process, which has been demonstrated using a lab-scale uni-axial rotational molding process. In particular, a data-driven modeling and control framework is developed that is able to reject raw material variation and achieve product quality which is specified through constraints on quality variables. To this end, a subspace state-space model of the rotational molding process is first identified from historical data generated in the lab. This dynamic model predicts the evolution of the internal mold temperature for a given set of input move trajectory (heater and compressed air profiles). Further, this dynamic model is augmented with a linear least-squares based quality model, which relates its terminal (states) prediction with key quality variables. For the lab-scale process, the chosen quality variables are sinkhole area, ultrasonic spectra amplitude, impact energy and shear viscosity. The complete model is then deployed within a model-based control scheme that facilitates specifying on-spec products via limits on the quality variables. Further, this framework is demonstrated to be capable of rejecting raw material variability to achieve the desired specifications. To replicate raw material variability observed in practice, in this work, the raw material is obtained by blending the matrix resin with a resin of slightly different viscosity at varying weight fractions. Results obtained from experimental studies demonstrate the capability of the proposed model predictive control (MPC) in meeting process specifications and rejecting raw material variability.
Record ID
Keywords
batch process modeling and control, Model Predictive Control, polymer processing, rotational molding, subspace identification
Subject
Suggested Citation
Garg A, Abdulhussain HA, Mhaskar P, Thompson MR. Handling Constraints and Raw Material Variability in Rotomolding through Data-Driven Model Predictive Control. (2019). LAPSE:2019.1199
Author Affiliations
Garg A: Department of Chemical Engineering, McMaster University, Hamilton, ON L8S 4L8, Canada [ORCID]
Abdulhussain HA: Department of Chemical Engineering, McMaster University, Hamilton, ON L8S 4L8, Canada [ORCID]
Mhaskar P: Department of Chemical Engineering, McMaster University, Hamilton, ON L8S 4L8, Canada [ORCID]
Thompson MR: Department of Chemical Engineering, McMaster University, Hamilton, ON L8S 4L8, Canada [ORCID]
[Login] to see author email addresses.
Abdulhussain HA: Department of Chemical Engineering, McMaster University, Hamilton, ON L8S 4L8, Canada [ORCID]
Mhaskar P: Department of Chemical Engineering, McMaster University, Hamilton, ON L8S 4L8, Canada [ORCID]
Thompson MR: Department of Chemical Engineering, McMaster University, Hamilton, ON L8S 4L8, Canada [ORCID]
[Login] to see author email addresses.
Journal Name
Processes
Volume
7
Issue
9
Article Number
E610
Year
2019
Publication Date
2019-09-10
Published Version
ISSN
2227-9717
Version Comments
Original Submission
Other Meta
PII: pr7090610, Publication Type: Journal Article
Record Map
Published Article
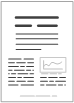
LAPSE:2019.1199
This Record
External Link
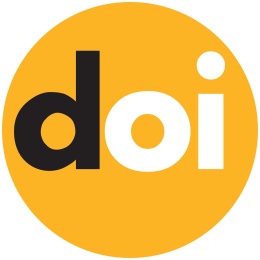
doi:10.3390/pr7090610
Publisher Version
Download
Meta
Record Statistics
Record Views
534
Version History
[v1] (Original Submission)
Nov 24, 2019
Verified by curator on
Nov 24, 2019
This Version Number
v1
Citations
Most Recent
This Version
URL Here
https://psecommunity.org/LAPSE:2019.1199
Original Submitter
Calvin Tsay
Links to Related Works