LAPSE:2018.0446
Published Article
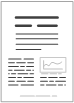
LAPSE:2018.0446
A Transformer Fault Diagnosis Model Using an Optimal Hybrid Dissolved Gas Analysis Features Subset with Improved Social Group Optimization-Support Vector Machine Classifier
September 19, 2018
Dissolved gas analysis (DGA) is widely used to detect the incipient fault of power transformers. However, the accuracy is greatly limited by selection of DGA features and performance of fault diagnostic model. This paper proposed a fault diagnostic method integrating feature selection and diagnostic model optimization. Firstly, this paper set up three feature sets with eight basic DGA gases, 28 DGA gas ratios and 36 hybrid DGA features, respectively. Then, to eliminate the interference of weak-relevant and irrelevant features, the genetic-algorithm-SVM-feature-screen (GA-SVM-FS) model was built to screen out the optimal hybrid DGA features subset (OHFS) from three feature sets. Next, using the OHFS as the input, the support vector machine (SVM) multi-classifier optimized by ISGOSVM (SVM classifier optimized by improved social group optimization) was built to diagnose fault types of transformers. Finally, the performance of OHFS and ISGOSVM diagnostic model was tested and compared with traditional DGA features and diagnostic models, respectively. The results show that the OHFS screened out is comprised of 14 features, including 12 gas ratios and two gases. The accuracy of OHFS is 3⁻30% higher than traditional DGA features, and the accuracy of ISGOSVM can increase by 3% to 14% compared with the SGOSVM (SVM classifier optimized by social group optimization), GASVM (SVM classifier optimized by genetic algorithm optimization), PSOSVM (SVM classifier optimized by particle swarm optimization), and SVM diagnostic models. The proposed approach integrating the OHFS with ISGOSVM achieves the highest accuracy of fault diagnose (92.86%).
Record ID
Keywords
dissolved gases, fault diagnosis, improved social group optimization, power transformers
Subject
Suggested Citation
Fang J, Zheng H, Liu J, Zhao J, Zhang Y, Wang K. A Transformer Fault Diagnosis Model Using an Optimal Hybrid Dissolved Gas Analysis Features Subset with Improved Social Group Optimization-Support Vector Machine Classifier. (2018). LAPSE:2018.0446
Author Affiliations
Fang J: Guangxi Key Laboratory of Power System Optimization and Energy Technology, Guangxi University, Nanning 530004, China [ORCID]
Zheng H: Guangxi Key Laboratory of Power System Optimization and Energy Technology, Guangxi University, Nanning 530004, China [ORCID]
Liu J: Guangxi Key Laboratory of Power System Optimization and Energy Technology, Guangxi University, Nanning 530004, China; National Demonstration Center for Experimental Electrical Engineering Education, Guangxi University, Nanning 530004, China [ORCID]
Zhao J: Department of Electrical and Computer Engineering & Computer Science, University of New Haven, West Haven, CT 06516, USA [ORCID]
Zhang Y: Guangxi Key Laboratory of Power System Optimization and Energy Technology, Guangxi University, Nanning 530004, China; National Demonstration Center for Experimental Electrical Engineering Education, Guangxi University, Nanning 530004, China [ORCID]
Wang K: China Electric Power Research Institute, Haidian District, Beijing 100192, China [ORCID]
[Login] to see author email addresses.
Zheng H: Guangxi Key Laboratory of Power System Optimization and Energy Technology, Guangxi University, Nanning 530004, China [ORCID]
Liu J: Guangxi Key Laboratory of Power System Optimization and Energy Technology, Guangxi University, Nanning 530004, China; National Demonstration Center for Experimental Electrical Engineering Education, Guangxi University, Nanning 530004, China [ORCID]
Zhao J: Department of Electrical and Computer Engineering & Computer Science, University of New Haven, West Haven, CT 06516, USA [ORCID]
Zhang Y: Guangxi Key Laboratory of Power System Optimization and Energy Technology, Guangxi University, Nanning 530004, China; National Demonstration Center for Experimental Electrical Engineering Education, Guangxi University, Nanning 530004, China [ORCID]
Wang K: China Electric Power Research Institute, Haidian District, Beijing 100192, China [ORCID]
[Login] to see author email addresses.
Journal Name
Energies
Volume
11
Issue
8
Article Number
E1922
Year
2018
Publication Date
2018-07-24
Published Version
ISSN
1996-1073
Version Comments
Original Submission
Other Meta
PII: en11081922, Publication Type: Journal Article
Record Map
Published Article
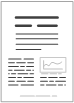
LAPSE:2018.0446
This Record
External Link
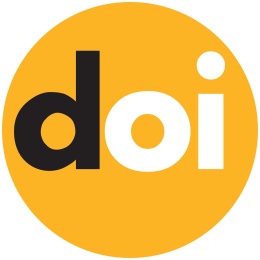
doi:10.3390/en11081922
Publisher Version
Download
Meta
Record Statistics
Record Views
575
Version History
[v1] (Original Submission)
Sep 19, 2018
Verified by curator on
Sep 19, 2018
This Version Number
v1
Citations
Most Recent
This Version
URL Here
https://psecommunity.org/LAPSE:2018.0446
Original Submitter
Calvin Tsay
Links to Related Works