LAPSE:2024.1278
Published Article
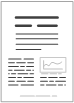
LAPSE:2024.1278
Centrifugal Pump Cavitation Fault Diagnosis Based on Feature-Level Multi-Source Information Fusion
June 21, 2024
In nuclear power systems, centrifugal pumps often need to operate under extreme conditions. However, accurately determining the cavitation status of centrifugal pumps under such extreme conditions is challenging. To improve the recognition accuracy of the three statuses of non-cavitation, incipient cavitation, and severe cavitation while improving the anti-interference capability of the monitoring system, this study extracted cavitation features from centrifugal pumps’ motor current and vibration signals under three different operational conditions. It fused the features using feature-level multi-source information fusion (MSIF) based on the backpropagation neural network (BPNN) or support vector machine (SVM) to construct a cavitation status recognition model and analyzed the results to compare with those of recognition without information fusion. The results show that, compared with one signal source, MSIF can significantly improve the recognition accuracy of cavitation statuses. Combined current and pump casing axial monitoring based on the BPNN is the optimal scheme, with an overall recognition accuracy of 97.3% for all operational conditions, compared to 73.9% for the single current signal and 89.3% for the single casing axial vibration signal. These research results can guide the monitoring of cavitation statuses in practical engineering, as well as timely intervention at incipient cavitations to reduce structural damage to centrifugal pumps and prolong service life.
Record ID
Keywords
backpropagation neural network, cavitation, centrifugal pump, feature-level multi-source information fusion, support vector machine
Subject
Suggested Citation
Song M, Zhi Y, An M, Xu W, Li G, Wang X. Centrifugal Pump Cavitation Fault Diagnosis Based on Feature-Level Multi-Source Information Fusion. (2024). LAPSE:2024.1278
Author Affiliations
Song M: LEO Group Hunan Pump Co., Ltd., Jiuhua Demonstration Zone, Xiangtan 411201, China
Zhi Y: China Nuclear Power Engineering Co., Ltd., Beijing 100840, China
An M: Research Center of Fluid Machinery Engineering and Technology, Zhenjiang 212013, China
Xu W: School of Energy and Power Engineering, Jiangsu University, Zhenjiang 212013, China
Li G: GongQing Institute of Science and Technology, Jiujiang 332020, China
Wang X: Research Center of Fluid Machinery Engineering and Technology, Zhenjiang 212013, China
Zhi Y: China Nuclear Power Engineering Co., Ltd., Beijing 100840, China
An M: Research Center of Fluid Machinery Engineering and Technology, Zhenjiang 212013, China
Xu W: School of Energy and Power Engineering, Jiangsu University, Zhenjiang 212013, China
Li G: GongQing Institute of Science and Technology, Jiujiang 332020, China
Wang X: Research Center of Fluid Machinery Engineering and Technology, Zhenjiang 212013, China
Journal Name
Processes
Volume
12
Issue
1
First Page
196
Year
2024
Publication Date
2024-01-16
Published Version
ISSN
2227-9717
Version Comments
Original Submission
Other Meta
PII: pr12010196, Publication Type: Journal Article
Record Map
Published Article
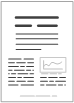
LAPSE:2024.1278
This Record
External Link
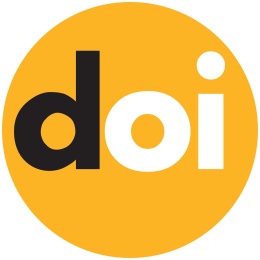
doi:10.3390/pr12010196
Publisher Version
Download
Meta
Record Statistics
Record Views
24
Version History
[v1] (Original Submission)
Jun 21, 2024
Verified by curator on
Jun 21, 2024
This Version Number
v1
Citations
Most Recent
This Version
URL Here
https://psecommunity.org/LAPSE:2024.1278
Original Submitter
Auto Uploader for LAPSE
Links to Related Works