LAPSE:2024.1139
Published Article
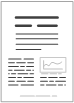
LAPSE:2024.1139
A Time−Frequency Residual Convolution Neural Network for the Fault Diagnosis of Rolling Bearings
June 21, 2024
A time−frequency residual convolution neural network (TFRCNN) was proposed to identify various rolling bearing fault types more efficiently. Three novel points about TFRCNN are presented as follows: First, by constructing a double-branch convolution network in the time domain and the frequency domain, the respective features in the time domain and the frequency domain were extracted to ensure the rich and complete feature representation of raw data sources. Second, specific residual structures were designed to prevent learning degradation of the deep network, and global average pooling was adopted to improve the network’s sparsity. Third, TFRCNN was better than the other models in terms of prediction accuracy, robustness, generalization ability, and convergence. The experimental results demonstrate that the prediction accuracy rate of TFRCNN, trained using mixing load data, reached 98.88 to 99.92% after optimizing the initial learning rate and choosing the optimizer and loss function. It was verified that TFRCNN can adaptively learn to extract deep fault features, accurately identify bearing fault conditions, and overcome the limitations of classical shallow feature extraction and classification methods, as well as common convolution neural networks. Hence, this investigation revealed TFRCNN’s potential for bearing fault diagnosis in practical engineering applications.
Record ID
Keywords
deep learning, double branch, fault diagnosis, generalization ability, prediction accuracy, robustness, rolling bearings
Subject
Suggested Citation
Wu C, Jiang R, Wu X, Zhong C, Huang C. A Time−Frequency Residual Convolution Neural Network for the Fault Diagnosis of Rolling Bearings. (2024). LAPSE:2024.1139
Author Affiliations
Wu C: School of Mechanical Engineering, Hunan Institute of Engineering, Xiangtan 411104, China; Hunan Provincial Engineering Laboratory of Wind Power Operation, Maintenance and Testing, Hunan Institute of Engineering, Xiangtan 411104, China; Hunan Provincial Ke
Jiang R: School of Mechanical Engineering, Hunan Institute of Engineering, Xiangtan 411104, China
Wu X: School of Mechanical Engineering, Hunan Institute of Engineering, Xiangtan 411104, China
Zhong C: School of Mechanical Engineering, Hunan Institute of Engineering, Xiangtan 411104, China
Huang C: School of Mechanical Engineering, Hunan Institute of Engineering, Xiangtan 411104, China
Jiang R: School of Mechanical Engineering, Hunan Institute of Engineering, Xiangtan 411104, China
Wu X: School of Mechanical Engineering, Hunan Institute of Engineering, Xiangtan 411104, China
Zhong C: School of Mechanical Engineering, Hunan Institute of Engineering, Xiangtan 411104, China
Huang C: School of Mechanical Engineering, Hunan Institute of Engineering, Xiangtan 411104, China
Journal Name
Processes
Volume
12
Issue
1
First Page
54
Year
2023
Publication Date
2023-12-25
Published Version
ISSN
2227-9717
Version Comments
Original Submission
Other Meta
PII: pr12010054, Publication Type: Journal Article
Record Map
Published Article
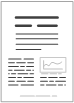
LAPSE:2024.1139
This Record
External Link
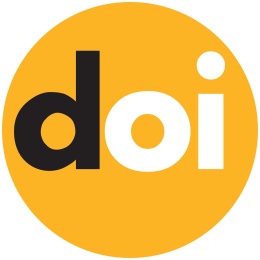
doi:10.3390/pr12010054
Publisher Version
Download
Meta
Record Statistics
Record Views
19
Version History
[v1] (Original Submission)
Jun 21, 2024
Verified by curator on
Jun 21, 2024
This Version Number
v1
Citations
Most Recent
This Version
URL Here
https://psecommunity.org/LAPSE:2024.1139
Original Submitter
Auto Uploader for LAPSE
Links to Related Works