LAPSE:2024.1029v1
Published Article
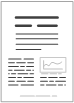
LAPSE:2024.1029v1
Research on a Small-Sample Fault Diagnosis Method for UAV Engines Based on an MSSST and ACS-BPNN Optimized Deep Convolutional Network
June 7, 2024
Regarding the difficulty of extracting fault information in the faulty status of UAV (unmanned aerial vehicle) engines and the high time cost and large data requirement of the existing deep learning fault diagnosis algorithms with many training parameters, in this paper, a small-sample transfer learning fault diagnosis algorithm is proposed. First, vibration signals under the engine fault status are converted into a two-dimensional time-frequency map by multiple simultaneous squeezing S-transform (MSSST), which reduces the randomness of manually extracted features. Second, to address the problems of slow network model training and large data sample requirement, a transfer diagnosis strategy using the fine-tuned time-frequency map samples as the pre-training model of the ResNet-18 convolutional neural network is proposed. In addition, in order to improve the training effect of the network model, an agent model is introduced to optimize the hyperparameter network autonomously. Finally, experiments show that the algorithm proposed in this paper can obtain high classification accuracy in fault diagnosis of UAV engines compared to other commonly used methods, with a classification accuracy of faults as high as 97.1751%; in addition, we show that it maintains a very stable small-sample migratory learning capability under this condition.
Record ID
Keywords
fault diagnosis, hyperparameter optimization, small sample, Surrogate Model, transfer learning
Subject
Suggested Citation
Li S, Liu Z, Yan Y, Han K, Han Y, Miao X, Cheng Z, Ma S. Research on a Small-Sample Fault Diagnosis Method for UAV Engines Based on an MSSST and ACS-BPNN Optimized Deep Convolutional Network. (2024). LAPSE:2024.1029v1
Author Affiliations
Li S: Shijiazhuang Campus, Army Engineering University of PLA, Shijiazhuang 050003, China
Liu Z: Shijiazhuang Campus, Army Engineering University of PLA, Shijiazhuang 050003, China [ORCID]
Yan Y: Shijiazhuang Campus, Army Engineering University of PLA, Shijiazhuang 050003, China
Han K: Shijiazhuang Campus, Army Engineering University of PLA, Shijiazhuang 050003, China
Han Y: Shijiazhuang Campus, Army Engineering University of PLA, Shijiazhuang 050003, China [ORCID]
Miao X: Armed Police Beijing Municipal Command Sixth Detachment on Duty, Beijing 100073, China
Cheng Z: Shijiazhuang Campus, Army Engineering University of PLA, Shijiazhuang 050003, China [ORCID]
Ma S: Shijiazhuang Division of PLAA Infantry Academy, Shijiazhuang 050003, China
Liu Z: Shijiazhuang Campus, Army Engineering University of PLA, Shijiazhuang 050003, China [ORCID]
Yan Y: Shijiazhuang Campus, Army Engineering University of PLA, Shijiazhuang 050003, China
Han K: Shijiazhuang Campus, Army Engineering University of PLA, Shijiazhuang 050003, China
Han Y: Shijiazhuang Campus, Army Engineering University of PLA, Shijiazhuang 050003, China [ORCID]
Miao X: Armed Police Beijing Municipal Command Sixth Detachment on Duty, Beijing 100073, China
Cheng Z: Shijiazhuang Campus, Army Engineering University of PLA, Shijiazhuang 050003, China [ORCID]
Ma S: Shijiazhuang Division of PLAA Infantry Academy, Shijiazhuang 050003, China
Journal Name
Processes
Volume
12
Issue
2
First Page
367
Year
2024
Publication Date
2024-02-10
Published Version
ISSN
2227-9717
Version Comments
Original Submission
Other Meta
PII: pr12020367, Publication Type: Journal Article
Record Map
Published Article
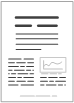
LAPSE:2024.1029v1
This Record
External Link
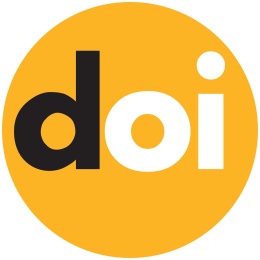
doi:10.3390/pr12020367
Publisher Version
Download
Meta
Record Statistics
Record Views
86
Version History
[v1] (Original Submission)
Jun 7, 2024
Verified by curator on
Jun 7, 2024
This Version Number
v1
Citations
Most Recent
This Version
URL Here
https://psecommunity.org/LAPSE:2024.1029v1
Original Submitter
Auto Uploader for LAPSE
Links to Related Works