LAPSE:2024.0321
Published Article
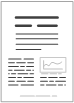
LAPSE:2024.0321
Novel Triplet Loss-Based Domain Generalization Network for Bearing Fault Diagnosis with Unseen Load Condition
June 5, 2024
In the real industrial manufacturing process, due to the constantly changing operational loads of equipment, it is difficult to collect data from all load conditions as the source domain signal for fault diagnosis. Therefore, the appearance of unseen load vibration signals in the target domain presents a challenge and research hotspot in fault diagnosis. This paper proposes a triplet loss-based domain generalization network (TL-DGN) and then applies it to an unseen domain bearing fault diagnosis. TL-DGN first utilizes a feature extractor to construct a multi-source domain classification loss. Furthermore, it measures the distance between class data from different domains using triplet loss. The introduced triplet loss can narrow the distance between samples of the same class in the feature space and widen the distance between samples of different classes based on the action of the cross-entropy loss function. It can reduce the dependency of the classification boundary on bearing operational loads, resulting in a more generalized classification model. Finally, two comparative experiments with fault diagnosis models without triplet loss and other classification models demonstrate that the proposed model achieves superior fault diagnosis performance.
Record ID
Keywords
domain generalization, fault diagnosis, triplet loss, unseen domain
Subject
Suggested Citation
Shen B, Zhang M, Yao L, Song Z. Novel Triplet Loss-Based Domain Generalization Network for Bearing Fault Diagnosis with Unseen Load Condition. (2024). LAPSE:2024.0321
Author Affiliations
Shen B: School of Mathematics, Hangzhou Normal University, Hangzhou 311121, China [ORCID]
Zhang M: State Key Laboratory of Industrial Control Technology, The College of Control Science and Engineering, Zhejiang University, Hangzhou 310027, China
Yao L: School of Mathematics, Hangzhou Normal University, Hangzhou 311121, China [ORCID]
Song Z: State Key Laboratory of Industrial Control Technology, The College of Control Science and Engineering, Zhejiang University, Hangzhou 310027, China
Zhang M: State Key Laboratory of Industrial Control Technology, The College of Control Science and Engineering, Zhejiang University, Hangzhou 310027, China
Yao L: School of Mathematics, Hangzhou Normal University, Hangzhou 311121, China [ORCID]
Song Z: State Key Laboratory of Industrial Control Technology, The College of Control Science and Engineering, Zhejiang University, Hangzhou 310027, China
Journal Name
Processes
Volume
12
Issue
5
First Page
882
Year
2024
Publication Date
2024-04-26
Published Version
ISSN
2227-9717
Version Comments
Original Submission
Other Meta
PII: pr12050882, Publication Type: Journal Article
Record Map
Published Article
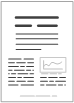
LAPSE:2024.0321
This Record
External Link
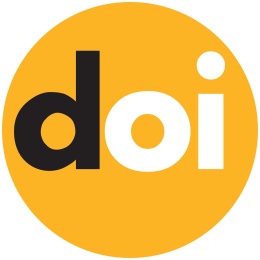
doi:10.3390/pr12050882
Publisher Version
Download
Meta
Record Statistics
Record Views
12
Version History
[v1] (Original Submission)
Jun 5, 2024
Verified by curator on
Jun 5, 2024
This Version Number
v1
Citations
Most Recent
This Version
URL Here
https://psecommunity.org/LAPSE:2024.0321
Original Submitter
Auto Uploader for LAPSE
Links to Related Works