LAPSE:2024.0266
Published Article
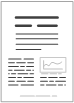
LAPSE:2024.0266
The Analysis and Rapid Non-Destructive Evaluation of Yongchuan Xiuya Quality Based on NIRS Combined with Machine Learning Methods
February 19, 2024
This paper attempts to analyze and assess Yongchuan Xiuya tea quality quickly, accurately, and digitally. The sensory evaluation method was first used to assess Yongchuan Xiuya tea quality, and then near infrared spectroscopy (NIRS) was obtained, and standard methods were applied to the testing of the chemical components. Next, principal component analysis (PCA) and the correlation coefficient method were used to comprehensively screen out the representative components. Finally, NIRS combined with partial least squares regression (PLSR) and back propagation artificial neural network (BP-ANN) methods were applied to build quality evaluation models for Yongchuan Xiuya tea, respectively, and external samples were employed to examine the practical application results of the best model. The cumulative variance contribution rate of the first three principal components of the ingredients in tea was 97.73%. Seven components closely related to tea quality were screened out, namely, amino acids, total catechin, epigallocatechin gallate (EGCG), tea polyphenols, water extracts, epicatechin gallate (ECG), and epigallocatechin (EGC) (p < 0.01). Between the two models established to predict the tea quality, the model built by the PLS method had the better results, whose coefficient of determination of prediction (Rp2) and root mean square error of prediction (RMSEP) were 0.7955 and 1.2263, respectively, and the best results were obtained by the nonlinear BP-ANN model, whose Rp2 and RMSEP were 0.9315 and 0.6787, respectively. The 10 external Yongchuan Xiuya samples were employed to test the best BP-ANN model, and the results of R2 and RMSEP were 0.9579 and 0.6086, respectively, meaning that the model has good robustness. Therefore, the model established by NIRS combined with the BP-ANN method can be used to assess Yongchuan Xiuya tea quality rapidly, accurately, and digitally, and it can also provide new ideas and methods for evaluating the quality of other teas.
Record ID
Keywords
artificial neural network, near infrared spectroscopy, principal component analysis, quality evaluation, Yongchuan Xiuya
Subject
Suggested Citation
Zang Y, Wang J, Wu X, Chang R, Wang Y, Luo H, Zhong Y, Wu Q, Chen Z, Deng M. The Analysis and Rapid Non-Destructive Evaluation of Yongchuan Xiuya Quality Based on NIRS Combined with Machine Learning Methods. (2024). LAPSE:2024.0266
Author Affiliations
Zang Y: Institute of Tea, Chongqing Academy of Agricultural Sciences, Chongqing 402160, China
Wang J: Institute of Tea, Chongqing Academy of Agricultural Sciences, Chongqing 402160, China [ORCID]
Wu X: Institute of Tea, Chongqing Academy of Agricultural Sciences, Chongqing 402160, China
Chang R: Institute of Tea, Chongqing Academy of Agricultural Sciences, Chongqing 402160, China
Wang Y: Institute of Tea, Chongqing Academy of Agricultural Sciences, Chongqing 402160, China
Luo H: Institute of Tea, Chongqing Academy of Agricultural Sciences, Chongqing 402160, China
Zhong Y: Institute of Tea, Chongqing Academy of Agricultural Sciences, Chongqing 402160, China
Wu Q: Institute of Tea, Chongqing Academy of Agricultural Sciences, Chongqing 402160, China
Chen Z: Institute of Tea, Chongqing Academy of Agricultural Sciences, Chongqing 402160, China
Deng M: Institute of Tea, Chongqing Academy of Agricultural Sciences, Chongqing 402160, China
Wang J: Institute of Tea, Chongqing Academy of Agricultural Sciences, Chongqing 402160, China [ORCID]
Wu X: Institute of Tea, Chongqing Academy of Agricultural Sciences, Chongqing 402160, China
Chang R: Institute of Tea, Chongqing Academy of Agricultural Sciences, Chongqing 402160, China
Wang Y: Institute of Tea, Chongqing Academy of Agricultural Sciences, Chongqing 402160, China
Luo H: Institute of Tea, Chongqing Academy of Agricultural Sciences, Chongqing 402160, China
Zhong Y: Institute of Tea, Chongqing Academy of Agricultural Sciences, Chongqing 402160, China
Wu Q: Institute of Tea, Chongqing Academy of Agricultural Sciences, Chongqing 402160, China
Chen Z: Institute of Tea, Chongqing Academy of Agricultural Sciences, Chongqing 402160, China
Deng M: Institute of Tea, Chongqing Academy of Agricultural Sciences, Chongqing 402160, China
Journal Name
Processes
Volume
11
Issue
9
First Page
2809
Year
2023
Publication Date
2023-09-21
Published Version
ISSN
2227-9717
Version Comments
Original Submission
Other Meta
PII: pr11092809, Publication Type: Journal Article
Record Map
Published Article
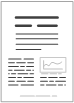
LAPSE:2024.0266
This Record
External Link
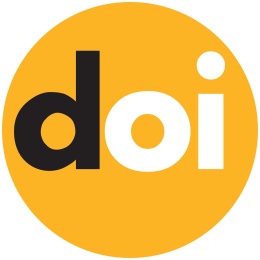
doi:10.3390/pr11092809
Publisher Version
Download
Meta
Record Statistics
Record Views
57
Version History
[v1] (Original Submission)
Feb 19, 2024
Verified by curator on
Feb 19, 2024
This Version Number
v1
Citations
Most Recent
This Version
URL Here
https://psecommunity.org/LAPSE:2024.0266
Original Submitter
Calvin Tsay
Links to Related Works