LAPSE:2024.0230
Published Article
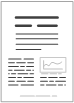
LAPSE:2024.0230
Advancing Fault Prediction: A Comparative Study between LSTM and Spiking Neural Networks
February 10, 2024
Predicting system faults is critical to improving productivity, reducing costs, and enforcing safety in industrial processes. Yet, traditional methodologies frequently falter due to the intricate nature of the task. This research presents a novel use of spiking neural networks (SNNs) in anticipating faults in syntactical time series, utilizing the generalized stochastic Petri net (GSPN) model. The inherent ability of SNNs to process both time and space aspects of data positions them as a prime instrument for this endeavor. A comparative evaluation with long short-term memory (LSTM) networks suggests that SNNs offer comparable robustness and performance.
Record ID
Keywords
generalized stochastic Petri net (GSPN), industrial processes, LSTM networks, spiking neural networks (SNNs), system fault prediction
Suggested Citation
Souza de Abreu R, Silva I, Nunes YT, Moioli RC, Guedes LA. Advancing Fault Prediction: A Comparative Study between LSTM and Spiking Neural Networks. (2024). LAPSE:2024.0230
Author Affiliations
Souza de Abreu R: Postgraduate Program in Electrical and Computer Engineering, Technology Center, Federal University of Rio Grande do Norte, Natal 59078-970, Brazil [ORCID]
Silva I: Postgraduate Program in Electrical and Computer Engineering, Technology Center, Federal University of Rio Grande do Norte, Natal 59078-970, Brazil [ORCID]
Nunes YT: Postgraduate Program in Electrical and Computer Engineering, Technology Center, Federal University of Rio Grande do Norte, Natal 59078-970, Brazil [ORCID]
Moioli RC: Bioinformatics Multidisciplinary Environment, Digital Metropolis Institute, Federal University of Rio Grande do Norte, Natal 59078-970, Brazil [ORCID]
Guedes LA: Postgraduate Program in Electrical and Computer Engineering, Technology Center, Federal University of Rio Grande do Norte, Natal 59078-970, Brazil [ORCID]
Silva I: Postgraduate Program in Electrical and Computer Engineering, Technology Center, Federal University of Rio Grande do Norte, Natal 59078-970, Brazil [ORCID]
Nunes YT: Postgraduate Program in Electrical and Computer Engineering, Technology Center, Federal University of Rio Grande do Norte, Natal 59078-970, Brazil [ORCID]
Moioli RC: Bioinformatics Multidisciplinary Environment, Digital Metropolis Institute, Federal University of Rio Grande do Norte, Natal 59078-970, Brazil [ORCID]
Guedes LA: Postgraduate Program in Electrical and Computer Engineering, Technology Center, Federal University of Rio Grande do Norte, Natal 59078-970, Brazil [ORCID]
Journal Name
Processes
Volume
11
Issue
9
First Page
2772
Year
2023
Publication Date
2023-09-16
Published Version
ISSN
2227-9717
Version Comments
Original Submission
Other Meta
PII: pr11092772, Publication Type: Journal Article
Record Map
Published Article
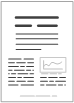
LAPSE:2024.0230
This Record
External Link
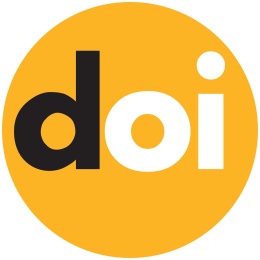
doi:10.3390/pr11092772
Publisher Version
Download
Meta
Record Statistics
Record Views
55
Version History
[v1] (Original Submission)
Feb 10, 2024
Verified by curator on
Feb 10, 2024
This Version Number
v1
Citations
Most Recent
This Version
URL Here
https://psecommunity.org/LAPSE:2024.0230
Original Submitter
Calvin Tsay
Links to Related Works