LAPSE:2024.0131
Published Article
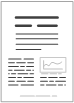
LAPSE:2024.0131
Classification and Evaluation of Tight Sandstone Reservoirs Based on MK-SVM
January 12, 2024
It is difficult to determine the main microscopic factors controlling reservoir quality due to the strong microscopic heterogeneity of tight sandstone reservoirs, which also makes it difficult to distinguish dominant reservoirs. At the same time, there are fewer experimental samples available, and data collected from relevant research are thus worth paying attention to. In this study, based on the experimental results of high-pressure mercury injection of 25 rock samples from Chang 6 reservoir in the Wuqi area, Lasso dimensionality reduction was used to reduce the dimensionality of 14 characteristic parameters to 6, which characterize the microscopic pore structure, while a combination of different kernel functions was used to construct the multi-kernel function of the multi-kernel model to be determined. A multi-kernel support vector machine (MK-SVM) model was established for unsupervised learning of microscopic pore structure characteristic parameters that affect reservoir quality. By optimizing the hyperparameters of the model and obtaining the optimal decision function, the tight sandstone reservoirs were classified into classes I, II and III, and the classification results were verified. The results show that the accuracy of the proposed reservoir classification method is as high as 86%, which can effectively reduce the time loss and save labor costs. It provides an effective method for the comprehensive evaluation of tight sandstone reservoirs.
Record ID
Keywords
high-pressure mercury compression, lasso dimensionality reduction, MK-SVM model, reservoir classification, tight sandstone reservoirs
Subject
Suggested Citation
Lu X, Xing X, Hu K, Zhou B. Classification and Evaluation of Tight Sandstone Reservoirs Based on MK-SVM. (2024). LAPSE:2024.0131
Author Affiliations
Lu X: College of Sciences, Xi’an Shiyou University, Xi’an 710065, China [ORCID]
Xing X: College of Mechanical Engineering, Xi’an Shiyou University, Xi’an 710065, China; Institute of Offshore Petroleum Engineering, Shanghai Offshore Oil & Gas Company, SINOPEC, Shanghai 200120, China
Hu K: The 11th Oil Production Plant of Petro China Changqing Oilfield Company, Qingyang 745000, China
Zhou B: The 10th Oil Production Plant of Petro China Changqing Oilfield Company, Qingyang 745000, China
Xing X: College of Mechanical Engineering, Xi’an Shiyou University, Xi’an 710065, China; Institute of Offshore Petroleum Engineering, Shanghai Offshore Oil & Gas Company, SINOPEC, Shanghai 200120, China
Hu K: The 11th Oil Production Plant of Petro China Changqing Oilfield Company, Qingyang 745000, China
Zhou B: The 10th Oil Production Plant of Petro China Changqing Oilfield Company, Qingyang 745000, China
Journal Name
Processes
Volume
11
Issue
9
First Page
2678
Year
2023
Publication Date
2023-09-06
Published Version
ISSN
2227-9717
Version Comments
Original Submission
Other Meta
PII: pr11092678, Publication Type: Journal Article
Record Map
Published Article
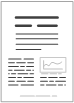
LAPSE:2024.0131
This Record
External Link
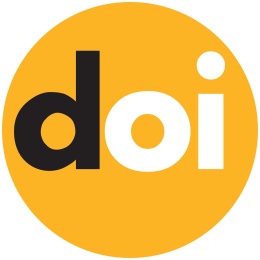
doi:10.3390/pr11092678
Publisher Version
Download
Meta
Record Statistics
Record Views
65
Version History
[v1] (Original Submission)
Jan 12, 2024
Verified by curator on
Jan 12, 2024
This Version Number
v1
Citations
Most Recent
This Version
URL Here
https://psecommunity.org/LAPSE:2024.0131
Original Submitter
Calvin Tsay
Links to Related Works