LAPSE:2024.0006
Published Article
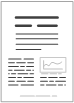
LAPSE:2024.0006
Ultra-Short-Term Load Forecasting for Customer-Level Integrated Energy Systems Based on Composite VTDS Models
January 5, 2024
A method is proposed to address the challenging issue of load prediction in user-level integrated energy systems (IESs) using a composite VTDS model. Firstly, an IES multi-dimensional load time series is decomposed into multiple intrinsic mode functions (IMFs) using variational mode decomposition (VMD). Then, each IMF, along with other influential features, is subjected to data dimensionality reduction and clustering denoising using t-distributed stochastic neighbor embedding (t-SNE) and fast density-based spatial clustering of applications with noise (FDBSCAN) to perform major feature selection. Subsequently, the reduced and denoised data are reconstructed, and a time-aware long short-term memory (T-LSTM) artificial neural network is employed to fill in missing data by incorporating time interval information. Finally, the selected multi-factor load time series is used as input into a support vector regression (SVR) model optimized using the quantum particle swarm optimization (QPSO) algorithm for load prediction. Using measured load data from a specific user-level IES at the Tempe campus of Arizona State University, USA, as a case study, a comparative analysis between the VTDS method and other approaches is conducted. The results demonstrate that the method proposed in this study achieved higher accuracy in short-term forecasting of the IES’s multiple loads.
Record ID
Keywords
feature selection, integrated energy systems, load forecasting, support vector regression, variational mode decomposition
Subject
Suggested Citation
Lu T, Hou S, Xu Y. Ultra-Short-Term Load Forecasting for Customer-Level Integrated Energy Systems Based on Composite VTDS Models. (2024). LAPSE:2024.0006
Author Affiliations
Lu T: School of Electrical and Electronic Engineering, North China Electric Power University, Baoding 071003, China
Hou S: School of Electrical and Electronic Engineering, North China Electric Power University, Baoding 071003, China
Xu Y: School of Electrical and Electronic Engineering, North China Electric Power University, Baoding 071003, China
Hou S: School of Electrical and Electronic Engineering, North China Electric Power University, Baoding 071003, China
Xu Y: School of Electrical and Electronic Engineering, North China Electric Power University, Baoding 071003, China
Journal Name
Processes
Volume
11
Issue
8
First Page
2461
Year
2023
Publication Date
2023-08-16
Published Version
ISSN
2227-9717
Version Comments
Original Submission
Other Meta
PII: pr11082461, Publication Type: Journal Article
Record Map
Published Article
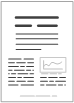
LAPSE:2024.0006
This Record
External Link
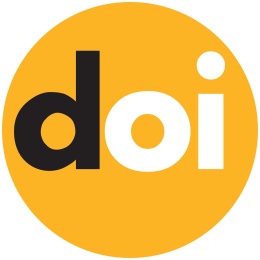
doi:10.3390/pr11082461
Publisher Version
Download
Meta
Record Statistics
Record Views
55
Version History
[v1] (Original Submission)
Jan 5, 2024
Verified by curator on
Jan 5, 2024
This Version Number
v1
Citations
Most Recent
This Version
URL Here
https://psecommunity.org/LAPSE:2024.0006
Original Submitter
Calvin Tsay
Links to Related Works