LAPSE:2023.4042
Published Article
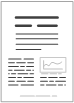
LAPSE:2023.4042
Impact of Sampling Technique on the Performance of Surrogate Models Generated with Artificial Neural Network (ANN): A Case Study for a Natural Gas Stabilization Unit
February 22, 2023
Data-driven models are essential tools for the development of surrogate models that can be used for the design, operation, and optimization of industrial processes. One approach of developing surrogate models is through the use of input−output data obtained from a process simulator. To enhance the model robustness, proper sampling techniques are required to cover the entire domain of the process variables uniformly. In the present work, Monte Carlo with pseudo-random samples as well as Latin hypercube samples and quasi-Monte Carlo samples with Hammersley Sequence Sampling (HSS) are generated. The sampled data obtained from the process simulator are fitted to neural networks for generating a surrogate model. An illustrative case study is solved to predict the gas stabilization unit performance. From the developed surrogate models to predict process data, it can be concluded that of the different sampling methods, Latin hypercube sampling and HSS have better performance than the pseudo-random sampling method for designing the surrogate model. This argument is based on the maximum absolute value, standard deviation, and the confidence interval for the relative average error as obtained from different sampling techniques.
Record ID
Keywords
process simulation, process systems engineering (PSE), sampling technique, stabilization unit, Surrogate Model
Suggested Citation
Ibrahim M, Al-Sobhi S, Mukherjee R, AlNouss A. Impact of Sampling Technique on the Performance of Surrogate Models Generated with Artificial Neural Network (ANN): A Case Study for a Natural Gas Stabilization Unit. (2023). LAPSE:2023.4042
Author Affiliations
Ibrahim M: Chemical Engineering Department, College of Engineering, Qatar University, Doha 2713, Qatar
Al-Sobhi S: Chemical Engineering Department, College of Engineering, Qatar University, Doha 2713, Qatar
Mukherjee R: Gas and Fuels Research Center, Texas A&M Engineering Experiment Station, College Station, TX 77843, USA
AlNouss A: Chemical Engineering Department, College of Engineering, Qatar University, Doha 2713, Qatar [ORCID]
[Login] to see author email addresses.
Al-Sobhi S: Chemical Engineering Department, College of Engineering, Qatar University, Doha 2713, Qatar
Mukherjee R: Gas and Fuels Research Center, Texas A&M Engineering Experiment Station, College Station, TX 77843, USA
AlNouss A: Chemical Engineering Department, College of Engineering, Qatar University, Doha 2713, Qatar [ORCID]
[Login] to see author email addresses.
Journal Name
Energies
Volume
12
Issue
10
Article Number
E1906
Year
2019
Publication Date
2019-05-18
Published Version
ISSN
1996-1073
Version Comments
Original Submission
Other Meta
PII: en12101906, Publication Type: Journal Article
Record Map
Published Article
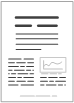
LAPSE:2023.4042
This Record
External Link
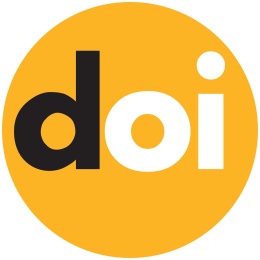
doi:10.3390/en12101906
Publisher Version
Download
Meta
Record Statistics
Record Views
100
Version History
[v1] (Original Submission)
Feb 22, 2023
Verified by curator on
Feb 22, 2023
This Version Number
v1
Citations
Most Recent
This Version
URL Here
https://psecommunity.org/LAPSE:2023.4042
Original Submitter
Auto Uploader for LAPSE
Links to Related Works