LAPSE:2023.36883
Published Article
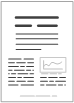
LAPSE:2023.36883
Research on Metallurgical Saw Blade Surface Defect Detection Algorithm Based on SC-YOLOv5
November 30, 2023
Under the background of intelligent manufacturing, in order to solve the complex problems of manual detection of metallurgical saw blade defects in enterprises, such as real-time detection, false detection, and the detection model being too large to deploy, a study on a metallurgical saw blade surface defect detection algorithm based on SC-YOLOv5 is proposed. Firstly, the SC network is built by integrating coordinate attention (CA) into the Shufflenet-V2 network, and the backbone network of YOLOv5 is replaced by the SC network to improve detection accuracy. Then, the SIOU loss function is used in the YOLOv5 prediction layer to solve the angle problem between the prediction frame and the real frame. Finally, in order to ensure both accuracy and speed, lightweight convolution (GSConv) is used to replace the ordinary convolution module. The experimental results show that the mAP@0.5 of the improved YOLOv5 model is 88.5%, and the parameter is 31.1M. Compared with the original YOLOv5 model, the calculation amount is reduced by 56.36%, and the map value is increased by 0.021. In addition, the overall performance of the improved SC-YOLOv5 model is better than that of the SSD and YOLOv3 target detection models. This method not only ensures the high detection rate of the model, but also significantly reduces the complexity of the model and the amount of parameter calculation. It meets the needs of deploying mobile terminals and provides an effective reference direction for applications in enterprises.
Record ID
Keywords
deep learning, defect detecting, lightweight, metallurgical saw blade, YOLOv5
Subject
Suggested Citation
Meng L, Cui X, Liu R, Zheng Z, Shao H, Liu J, Peng Y, Zheng L. Research on Metallurgical Saw Blade Surface Defect Detection Algorithm Based on SC-YOLOv5. (2023). LAPSE:2023.36883
Author Affiliations
Meng L: College of Mechanical Engineering, North China University of Science and Technology, Tangshan 063210, China
Cui X: College of Mechanical Engineering, North China University of Science and Technology, Tangshan 063210, China
Liu R: College of Metallurgy & Energy, North China University of Science and Technology, Tangshan 063210, China
Zheng Z: College of Mechanical Engineering, North China University of Science and Technology, Tangshan 063210, China [ORCID]
Shao H: Tangshan Metallurgical Saw Blade Co., Ltd., Tangshan 063000, China
Liu J: College of Mechanical Engineering, North China University of Science and Technology, Tangshan 063210, China
Peng Y: College of Mechanical Engineering, North China University of Science and Technology, Tangshan 063210, China
Zheng L: College of Mechanical Engineering, North China University of Science and Technology, Tangshan 063210, China
Cui X: College of Mechanical Engineering, North China University of Science and Technology, Tangshan 063210, China
Liu R: College of Metallurgy & Energy, North China University of Science and Technology, Tangshan 063210, China
Zheng Z: College of Mechanical Engineering, North China University of Science and Technology, Tangshan 063210, China [ORCID]
Shao H: Tangshan Metallurgical Saw Blade Co., Ltd., Tangshan 063000, China
Liu J: College of Mechanical Engineering, North China University of Science and Technology, Tangshan 063210, China
Peng Y: College of Mechanical Engineering, North China University of Science and Technology, Tangshan 063210, China
Zheng L: College of Mechanical Engineering, North China University of Science and Technology, Tangshan 063210, China
Journal Name
Processes
Volume
11
Issue
9
First Page
2564
Year
2023
Publication Date
2023-08-27
Published Version
ISSN
2227-9717
Version Comments
Original Submission
Other Meta
PII: pr11092564, Publication Type: Journal Article
Record Map
Published Article
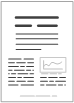
LAPSE:2023.36883
This Record
External Link
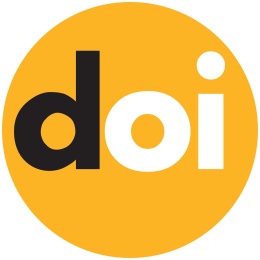
doi:10.3390/pr11092564
Publisher Version
Download
Meta
Record Statistics
Record Views
62
Version History
[v1] (Original Submission)
Nov 30, 2023
Verified by curator on
Nov 30, 2023
This Version Number
v1
Citations
Most Recent
This Version
URL Here
https://psecommunity.org/LAPSE:2023.36883
Original Submitter
Calvin Tsay
Links to Related Works