LAPSE:2023.36839
Published Article
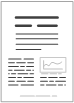
LAPSE:2023.36839
Explainable Machine Learning-Based Method for Fracturing Prediction of Horizontal Shale Oil Wells
November 30, 2023
Hydraulic fracturing is a crucial method in shale oil development, and predicting production after hydraulic fracturing is one of the challenges in shale oil development. Conventional methods for predicting production include analytical methods and numerical simulation methods, but these methods involve many parameters, have high uncertainty, and are time-consuming and costly. With the development of shale oil development, there are more and more sample data on the geological parameters, engineering parameters, and development parameters of shale oil hydraulic fracturing, making it possible to use machine learning methods to predict production after hydraulic fracturing. This article first analyzes the impact of different parameters on initial production and recoverable reserves based on field data from Chang-7 shale oil in the Ordos Basin of China. Then, using the Particle Swarm Optimization (PSO) algorithm and the Gradient Boosting Decision Tree (GBDT) algorithm, machine learning models for initial production and recoverable reserves are established. The Local Interpretable Model-Agnostic Explanations (LIME) and Shapley Additive exPlanations (SHAP) explanation methods are used to explain the models. The study found that initial production is highly correlated with parameters such as the number of fracturing stages and fracturing fluid volume, while recoverable reserves are significantly related to parameters such as well spacing, area, and reserver-controlled. The PSO-GBDT model established in this study has an accuracy of over 85% and can be used for production prediction and subsequent parameter optimization research. By comparing the LIME and SHAP local explanation methods, it is shown that different explanation methods can obtain reasonable and credible local explanation results. This article establishes a high-precision shale oil well production prediction model and two model interpretation methods, which could provide technical support for shale oil well production prediction and production analysis.
Record ID
Keywords
explainable algorithm, hydraulic fracturing horizontal wells, machine learning productivity prediction model, PSO-GBDT, shale oil
Subject
Suggested Citation
Liu X, Zhang T, Yang H, Qian S, Dong Z, Li W, Zou L, Liu Z, Wang Z, Zhang T, Lin K. Explainable Machine Learning-Based Method for Fracturing Prediction of Horizontal Shale Oil Wells. (2023). LAPSE:2023.36839
Author Affiliations
Liu X: Petrochina Changqing Oilfield Company, Xi’an 710021, China; State Key Laboratory of Oil and Gas Resources and Exploration, China University of Petroleum (Beijing), Beijing 102249, China
Zhang T: Ptroleum Engineering Department, Xi’an Shiyou University, Xi’an 710065, China [ORCID]
Yang H: Petrochina Changqing Oilfield Company, Xi’an 710021, China
Qian S: Ptroleum Engineering Department, Xi’an Shiyou University, Xi’an 710065, China [ORCID]
Dong Z: Ptroleum Engineering Department, Xi’an Shiyou University, Xi’an 710065, China
Li W: Ptroleum Engineering Department, Xi’an Shiyou University, Xi’an 710065, China [ORCID]
Zou L: Ptroleum Engineering Department, Xi’an Shiyou University, Xi’an 710065, China
Liu Z: Research Institute of Petroleum Exploration and Development, PetroChina, Beijing 100083, China
Wang Z: Research Institute of Petroleum Exploration and Development, PetroChina, Beijing 100083, China
Zhang T: State Key Laboratory of Oil and Gas Resources and Exploration, China University of Petroleum (Beijing), Beijing 102249, China
Lin K: State Key Laboratory of Oil and Gas Resources and Exploration, China University of Petroleum (Beijing), Beijing 102249, China
Zhang T: Ptroleum Engineering Department, Xi’an Shiyou University, Xi’an 710065, China [ORCID]
Yang H: Petrochina Changqing Oilfield Company, Xi’an 710021, China
Qian S: Ptroleum Engineering Department, Xi’an Shiyou University, Xi’an 710065, China [ORCID]
Dong Z: Ptroleum Engineering Department, Xi’an Shiyou University, Xi’an 710065, China
Li W: Ptroleum Engineering Department, Xi’an Shiyou University, Xi’an 710065, China [ORCID]
Zou L: Ptroleum Engineering Department, Xi’an Shiyou University, Xi’an 710065, China
Liu Z: Research Institute of Petroleum Exploration and Development, PetroChina, Beijing 100083, China
Wang Z: Research Institute of Petroleum Exploration and Development, PetroChina, Beijing 100083, China
Zhang T: State Key Laboratory of Oil and Gas Resources and Exploration, China University of Petroleum (Beijing), Beijing 102249, China
Lin K: State Key Laboratory of Oil and Gas Resources and Exploration, China University of Petroleum (Beijing), Beijing 102249, China
Journal Name
Processes
Volume
11
Issue
9
First Page
2520
Year
2023
Publication Date
2023-08-22
Published Version
ISSN
2227-9717
Version Comments
Original Submission
Other Meta
PII: pr11092520, Publication Type: Journal Article
Record Map
Published Article
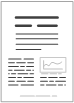
LAPSE:2023.36839
This Record
External Link
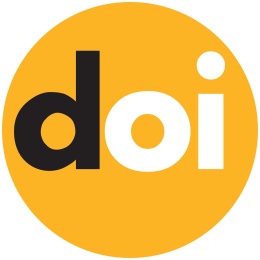
doi:10.3390/pr11092520
Publisher Version
Download
Meta
Record Statistics
Record Views
69
Version History
[v1] (Original Submission)
Nov 30, 2023
Verified by curator on
Nov 30, 2023
This Version Number
v1
Citations
Most Recent
This Version
URL Here
https://psecommunity.org/LAPSE:2023.36839
Original Submitter
Calvin Tsay
Links to Related Works