LAPSE:2023.36715
Published Article
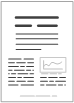
LAPSE:2023.36715
Predicting Shear Wave Velocity Using a Convolutional Neural Network and Dual-Constraint Calculation for Anisotropic Parameters Incorporating Compressional and Shear Wave Velocities
September 21, 2023
As the exploration of unconventional reservoirs progresses, characterizing challenging formations like tight sandstone becomes increasingly complex. Anisotropic parameters play a vital role in accurately characterizing these unconventional reservoirs. In light of this, this paper introduces an approach that uses a dual-constraint anisotropic rock physics model based on compressional and shear wave velocities. The proposed method aims to enhance the precision of anisotropic parameter calculations, thus improving the overall accuracy of reservoir characterization. The paper begins by applying a convolutional neural network (CNN) to predict shear wave velocity, effectively resolving the issue of incomplete shear wave logging data. Subsequently, an anisotropic rock physics model is developed specifically for tight sandstone. A comprehensive analysis is conducted to examine the influence of quartz, clay porosity aspect ratio, and fracture density on compressional and shear wave velocities. Trial calculations using the anisotropic model data demonstrated that the accuracy of calculating anisotropic parameters significantly improved when both compressional and transverse wave velocity constraints were taken into account, as opposed to relying solely on the compressional wave velocity constraint. Furthermore, the rationality of predicting anisotropic parameters using both the shear wave velocity predicted by the convolutional neural network and the measured compressional wave velocity was confirmed using the example of deep tight sandstone in the Junggar Basin.
Record ID
Keywords
anisotropic parameters, CNN, dual constraints encompassing both compressional and shear wave velocities, shear wave velocity prediction
Suggested Citation
Liu J, Gui Z, Gao G, Li Y, Wei Q, Liu Y. Predicting Shear Wave Velocity Using a Convolutional Neural Network and Dual-Constraint Calculation for Anisotropic Parameters Incorporating Compressional and Shear Wave Velocities. (2023). LAPSE:2023.36715
Author Affiliations
Liu J: Key Laboratory of Exploration Technologies for Oil and Gas Resources, Yangtze University, Ministry of Education, Wuhan 430100, China
Gui Z: Key Laboratory of Exploration Technologies for Oil and Gas Resources, Yangtze University, Ministry of Education, Wuhan 430100, China
Gao G: Key Laboratory of Exploration Technologies for Oil and Gas Resources, Yangtze University, Ministry of Education, Wuhan 430100, China
Li Y: Research Institute of Petroleum Exploration and Development, CNPC, Beijing 100083, China
Wei Q: Key Laboratory of Exploration Technologies for Oil and Gas Resources, Yangtze University, Ministry of Education, Wuhan 430100, China
Liu Y: Key Laboratory of Exploration Technologies for Oil and Gas Resources, Yangtze University, Ministry of Education, Wuhan 430100, China
Gui Z: Key Laboratory of Exploration Technologies for Oil and Gas Resources, Yangtze University, Ministry of Education, Wuhan 430100, China
Gao G: Key Laboratory of Exploration Technologies for Oil and Gas Resources, Yangtze University, Ministry of Education, Wuhan 430100, China
Li Y: Research Institute of Petroleum Exploration and Development, CNPC, Beijing 100083, China
Wei Q: Key Laboratory of Exploration Technologies for Oil and Gas Resources, Yangtze University, Ministry of Education, Wuhan 430100, China
Liu Y: Key Laboratory of Exploration Technologies for Oil and Gas Resources, Yangtze University, Ministry of Education, Wuhan 430100, China
Journal Name
Processes
Volume
11
Issue
8
First Page
2356
Year
2023
Publication Date
2023-08-05
Published Version
ISSN
2227-9717
Version Comments
Original Submission
Other Meta
PII: pr11082356, Publication Type: Journal Article
Record Map
Published Article
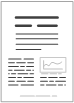
LAPSE:2023.36715
This Record
External Link
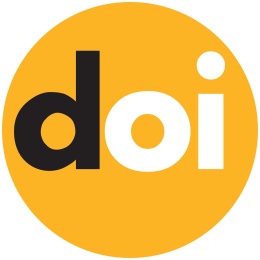
doi:10.3390/pr11082356
Publisher Version
Download
Meta
Record Statistics
Record Views
110
Version History
[v1] (Original Submission)
Sep 21, 2023
Verified by curator on
Sep 21, 2023
This Version Number
v1
Citations
Most Recent
This Version
URL Here
https://psecommunity.org/LAPSE:2023.36715
Original Submitter
Calvin Tsay
Links to Related Works