LAPSE:2023.36691
Published Article
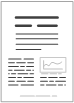
LAPSE:2023.36691
Remaining Useful Life Prediction for Lithium-Ion Batteries Based on a Hybrid Deep Learning Model
September 20, 2023
Lithium-ion batteries are widely utilized in various fields, including aerospace, new energy vehicles, energy storage systems, medical equipment, and security equipment, due to their high energy density, extended lifespan, and lightweight design. Precisely predicting the remaining useful life (RUL) of lithium batteries is crucial for ensuring the safe use of a device. In order to solve the problems of unstable prediction accuracy and difficultly modeling lithium-ion battery RUL with previous methods, this paper combines a channel attention (CA) mechanism and long short-term memory networks (LSTM) to propose a new hybrid CA-LSTM lithium-ion battery RUL prediction model. By incorporating a CA mechanism, the utilization of local features in situations where data are limited can be improved. Additionally, the CA mechanism can effectively mitigate the impact of battery capacity rebound on the model during lithium-ion battery charging and discharging cycles. In order to ensure the full validity of the experiments, this paper utilized the National Aeronautics and Space Administration (NASA) and the University of Maryland Center for Advanced Life Cycle Engineering (CALCE) lithium-ion battery datasets and different prediction starting points for model validation. The experimental results demonstrated that the hybrid CA-LSTM lithium-ion battery RUL prediction model proposed in this paper exhibited a strong predictive performance and was minimally influenced by the prediction starting point.
Record ID
Keywords
channel attention mechanism, lithium-ion batteries, long short-term memory, remaining useful life
Subject
Suggested Citation
Chen C, Wei J, Li Z. Remaining Useful Life Prediction for Lithium-Ion Batteries Based on a Hybrid Deep Learning Model. (2023). LAPSE:2023.36691
Author Affiliations
Chen C: College of Computer Science and Engineering, Sichuan University of Science and Engineering, Zigong 643000, China; Sichuan Key Provincial Research Base of Intelligent Tourism, Zigong 643000, China [ORCID]
Wei J: College of Computer Science and Engineering, Sichuan University of Science and Engineering, Zigong 643000, China [ORCID]
Li Z: College of Computer Science and Engineering, Sichuan University of Science and Engineering, Zigong 643000, China [ORCID]
Wei J: College of Computer Science and Engineering, Sichuan University of Science and Engineering, Zigong 643000, China [ORCID]
Li Z: College of Computer Science and Engineering, Sichuan University of Science and Engineering, Zigong 643000, China [ORCID]
Journal Name
Processes
Volume
11
Issue
8
First Page
2333
Year
2023
Publication Date
2023-08-03
Published Version
ISSN
2227-9717
Version Comments
Original Submission
Other Meta
PII: pr11082333, Publication Type: Journal Article
Record Map
Published Article
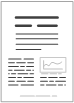
LAPSE:2023.36691
This Record
External Link
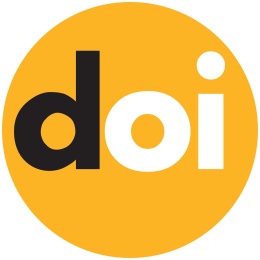
doi:10.3390/pr11082333
Publisher Version
Download
Meta
Record Statistics
Record Views
84
Version History
[v1] (Original Submission)
Sep 20, 2023
Verified by curator on
Sep 20, 2023
This Version Number
v1
Citations
Most Recent
This Version
URL Here
https://psecommunity.org/LAPSE:2023.36691
Original Submitter
Calvin Tsay
Links to Related Works