LAPSE:2023.36614
Published Article
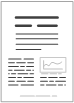
LAPSE:2023.36614
A Novel Cellular Network Traffic Prediction Algorithm Based on Graph Convolution Neural Networks and Long Short-Term Memory through Extraction of Spatial-Temporal Characteristics
September 20, 2023
In recent years, cellular communication systems have continued to develop in the direction of intelligence. The demand for cellular networks is increasing as they meet the public’s pursuit of a better life. Accurate prediction of cellular network traffic can help operators avoid wasting resources and improve management efficiency. Traditional prediction methods can no longer perfectly cope with the highly complex spatiotemporal relationships of the current cellular networks, and prediction methods based on deep learning are constantly growing. In this paper, a spatial-temporal parallel prediction model based on graph convolution combined with long and short-term memory networks (STP-GLN) is proposed to effectively capture spatial-temporal characteristics and to obtain accurate prediction results. STP-GLN is mainly composed of a spatial module and temporal module. Among them, the spatial module designs dynamic graph data based on the principle of spatial distance and spatial correlation. It uses a graph convolutional neural network to learn the spatial characteristics of cellular network graph data. The temporal module uses three time series based on the principle of temporal proximity and temporal periodicity. It uses three long and short-term memory networks to learn the temporal characteristics of three time series of cellular network data. Finally, the results learned from the two modules are fused with different weights to obtain the final prediction results. The mean absolute error (MAE), root mean square error (RMSE), and R-squared (R2) are used as the performance evaluation metrics of the model in this paper. The experimental results show that STP-GLN can more effectively capture the spatiotemporal characteristics of cellular network data; compared with the most advanced model in the comparison model on the real cellular traffic dataset in one cell, the RMSE can be improved about 81.7%, the MAE is improved about 82.7%, and the R2 is improved about 2.2%.
Record ID
Keywords
cellular network, graph convolutional neural networks, RMSE, short and long-term memory networks, traffic prediction
Subject
Suggested Citation
Chen G, Guo Y, Zeng Q, Zhang Y. A Novel Cellular Network Traffic Prediction Algorithm Based on Graph Convolution Neural Networks and Long Short-Term Memory through Extraction of Spatial-Temporal Characteristics. (2023). LAPSE:2023.36614
Author Affiliations
Chen G: College of Electronic and Information Engineering, Shandong University of Science and Technology, Qingdao 266590, China [ORCID]
Guo Y: College of Electronic and Information Engineering, Shandong University of Science and Technology, Qingdao 266590, China
Zeng Q: College of Electronic and Information Engineering, Shandong University of Science and Technology, Qingdao 266590, China
Zhang Y: School of Computing and Mathematical Sciences, University of Leicester, Leicester LE1 7RH, UK [ORCID]
Guo Y: College of Electronic and Information Engineering, Shandong University of Science and Technology, Qingdao 266590, China
Zeng Q: College of Electronic and Information Engineering, Shandong University of Science and Technology, Qingdao 266590, China
Zhang Y: School of Computing and Mathematical Sciences, University of Leicester, Leicester LE1 7RH, UK [ORCID]
Journal Name
Processes
Volume
11
Issue
8
First Page
2257
Year
2023
Publication Date
2023-07-26
Published Version
ISSN
2227-9717
Version Comments
Original Submission
Other Meta
PII: pr11082257, Publication Type: Journal Article
Record Map
Published Article
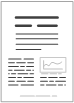
LAPSE:2023.36614
This Record
External Link
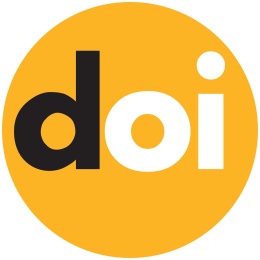
doi:10.3390/pr11082257
Publisher Version
Download
Meta
Record Statistics
Record Views
86
Version History
[v1] (Original Submission)
Sep 20, 2023
Verified by curator on
Sep 20, 2023
This Version Number
v1
Citations
Most Recent
This Version
URL Here
https://psecommunity.org/LAPSE:2023.36614
Original Submitter
Calvin Tsay
Links to Related Works